I have to do a project that tries to scan the shape of the vehicles and detect what type of vehicle it is , the scanning will performed with a sensors called “vehicle scanner” they are just 50 beams of lights, each beam with receptor and emission as it is shown in the picture:
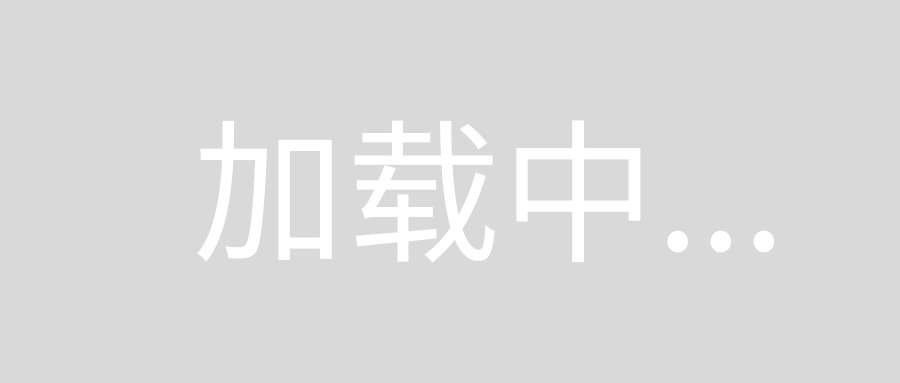
I get from the sensors the raw sate of each beam (block or unblock) and with that continuous scanning we can create a probably very low res image of the vehicle.
My question is what algorithms/technique I can use to detect and identify the shape of the vehicle, we want to count the wheels, and if we can, try to identify if that shape is a car or a truck or pickup etc., at least we want to count the wheels.
I am considering training a neural network, but perhaps could be a simpler approach for this kind of pattern detection that I can use and I am unaware of.
Any other suggestion/advice will be much appreciated
A standard neural network would be a reasonable choice and would work, however a convolutional neural network (CNN) would probably be the best choice (see this for a quick explanation). CNNs are great for image recognition since their sparse connectivity allows for spatially local correlation (i.e. they take into account the relationships between inputs within close proximity to one another) meaning that they generalise to new data-sets more effectively than standard neural nets, and are also faster to train.
In order to detect the number of wheels, one could split the low res input into a number of overlapping 'wheel sized' patches, then use each patch as input to a CNN which has been trained to detect wheels. Since there is the possibility of the CNN returning true for multiple patches around the same wheel, a proximity checker would need to be implemented so that each of the local 'true' patches causes only a single incrementation of the total counter. This could be done by identifying the local patch with the highest output node activation, and by preventing any other patch within the circumference of this patch from affecting the total counter.
Identifying the shape as a car or truck would in fact be a simpler task as the entire image could be fed to a CNN trained on a selection of pre-classified vehicle images. It would be possible to work around the squashing/stretching effects of speed by augmenting the training datasets with random squashing/stretching deformations. For advise on how to setup the parameters in a CNN, see how do you decide the parameters of a convolutional neural network for image classification.
As proof of how effective CNNs are, take a look at the results of the Large Scale Visual Recognition Challenge 2012 (LSVRC). LSVRC was an image classification competition where competitors competed to achieve the lowest classification error on an arbitrary selection of 256x256 images. The winning network, named Supervision, achieved almost half the error of its closes competitor by using the CNN model. CNNs also hold the record for the highest accuracy on many text recognition tasks, for example the MNIST digit recognition task in which the model scored an accuracy of 99.8% - an accuracy which rivals human recognition rates.
You should be able to get vehicle, height (to a max height), perhaps number of wheels, location/shape of windows (if the beams go through the windows) and the general shape.
You can probably just have a template (or a few templates) for what the side profile of a car, truck, van ect look like. You can then stretch each templates to the dimensions measure and subtract the recorded shape from the template shape. The template with the least difference is the closest match. This can be improved by allowing the shape to be more variable. For example, the height of the hood could be moved up or down to some degree based on min/max recorded ratios of hood height to roof height. If you have a collection of such ratios (or actual recorded values if you find them online) and templates, then you should be able to do well enough. You could get these ratios simply by analyzing a number of vehicle photos.
This should work fairly well overall if you have good, representative templates and aren't trying to be too specific as to what the vehicle is. For example, finding templates that you can use to tell the difference between an crossover and a van might be difficult, given how your system is stated to work, but should work fine if you allow for a bit of leeway as to what a crossover is classified as.
Edit:
Actually, you could use a single template and just have a few adjustable points (up to around 10 such points), the configuration of which could be used to classify the vehicle. A few examples:
- Start of the hood
- Hood/windshield intersection
- Roof/windshield intersection
- Tire/body intersection (2 such points for each tire)
The result would be a blocky but fairly accurate vehicle shape. Roughly where those points are and if they exist at all should be useful for telling vehicle type. Although, having fixed templates would be much simpler and if say a van is listed as a truck, you could probably use that van as an additional template for a van.
You main concern would be the speed of the vehicle as a faster vehicle will give you lesser numbers of observation which would be inaccurate. Following is way to check :-
Algorithm :-
1. Height is accurate metric to check as it is not affected by speed
2. get a median of all the heights you get , that would be close to exact height.
3. you can also evaluate the width which is not correct as speed can change.
4. ratio height/width can be checked.
5. there are certain ranges of height/width ratio for car,truck etc.
6. height can mostly classify between truck and cars.
7. ratio of height/width can be used to scale the image to correct range.
8. After scaling you might give that image to neural network which you trained.
9. train neural network with already gathered real life observations if you can.
10. May be you can also create a simulation by 3d modelling and animation.