Given a 2 dimensional array, where each row represents the position vector of a particle, how do I compute the mean square displacement efficiently (using FFT)?
The mean square displacement is defined as
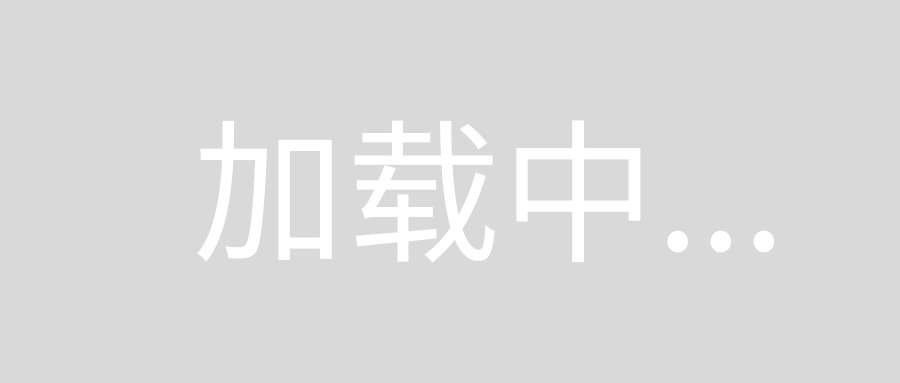
where r(m) is the position vector of row m, and N is the number of rows.
The following straight forward method for the msd works, but it is O(N**2) (I adapted the code from this stackoverflow answer by user morningsun)
def msd_straight_forward(r):
shifts = np.arange(len(r))
msds = np.zeros(shifts.size)
for i, shift in enumerate(shifts):
diffs = r[:-shift if shift else None] - r[shift:]
sqdist = np.square(diffs).sum(axis=1)
msds[i] = sqdist.mean()
return msds
However, we can make this code way faster using the FFT. The following consideration and the resulting algorithm are from this paper, I will just show how to implement it in python.
We can split the MSD in the following way
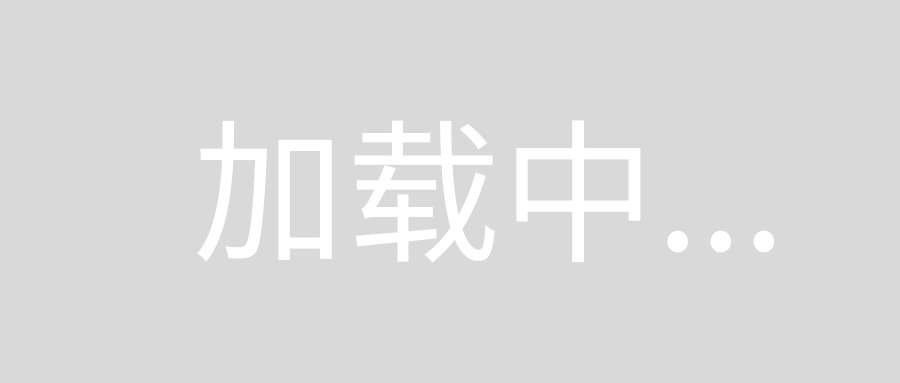
Thereby, S_2(m) is just the autocorrelation of the position. Note that in some textbooks S_2(m) is denoted as autocorrelation (convention A) and in some S_2(m)*(N-m) is denoted as autocorrelation (convention B).
By the Wiener–Khinchin theorem, the power spectral density (PSD) of a function is the Fourier transform of the autocorrelation.
This means we can compute a PSD of a signal and Fourier-invert it, to get the autocorrelation (in convention B). For discrete signals we get the cyclic autocorrelation.
However, by zero-padding the data, we can get the non-cyclic autocorrelation. The algorithm looks like this
def autocorrFFT(x):
N=len(x)
F = np.fft.fft(x, n=2*N) #2*N because of zero-padding
PSD = F * F.conjugate()
res = np.fft.ifft(PSD)
res= (res[:N]).real #now we have the autocorrelation in convention B
n=N*np.ones(N)-np.arange(0,N) #divide res(m) by (N-m)
return res/n #this is the autocorrelation in convention A
For the term S_1(m), we exploit the fact, that a recursive relation for (N-m)*S_1(m) can be found (This is explained in this paper in section 4.2).
We define
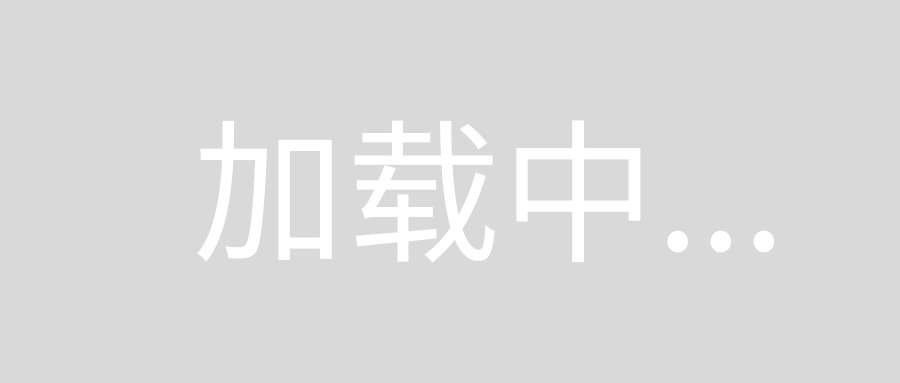
And find S_1(m) via
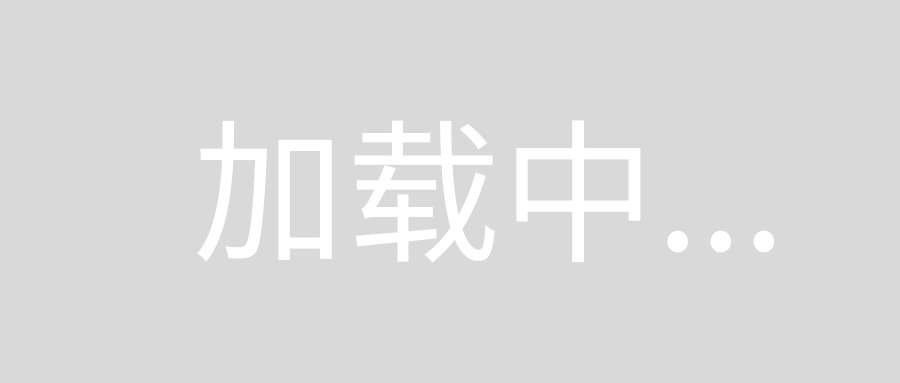
This yields the following code for the mean square displacement
def msd_fft(r):
N=len(r)
D=np.square(r).sum(axis=1)
D=np.append(D,0)
S2=sum([autocorrFFT(r[:, i]) for i in range(r.shape[1])])
Q=2*D.sum()
S1=np.zeros(N)
for m in range(N):
Q=Q-D[m-1]-D[N-m]
S1[m]=Q/(N-m)
return S1-2*S2
You can compare msd_straight_forward() and msd_fft() and will find that they yield the same results, though msd_fft() is way faster for large N
A small benchmark: Generate a trajectory with
r = np.cumsum(np.random.choice([-1., 0., 1.], size=(N, 3)), axis=0)
For N=100.000, we get
$ %timeit msd_straight_forward(r)
1 loops, best of 3: 2min 1s per loop
$ %timeit msd_fft(r)
10 loops, best of 3: 253 ms per loop
Using numpy.cumsum you can also avoid looping over range(N) in the S1 calculation:
sq = map(sum, map(np.square, r))
s1 = 2 * sum(sq) - np.cumsum(np.insert(sq[0:-1], 0, 0) + np.flip(np.append(sq[1:], 0), 0))