Following up from here: Converting a 1D array into a 2D class-based matrix in python
I want to draw ROC curves for each of my 46 classes. I have 300 test samples for which I've run my classifier to make a prediction.
y_test
is the true classes, and y_pred
is what my classifier predicted.
Here's my code:
from sklearn.metrics import confusion_matrix, roc_curve, auc
from sklearn.preprocessing import label_binarize
import numpy as np
y_test_bi = label_binarize(y_test, classes=[0,1,2,3,4,5,6,7,8,9,10,11,12,13,14,15,16,17,18, 19,20,21,2,23,24,25,26,27,28,29,30,31,32,33,34,35,36,37,38,3,40,41,42,43,44,45])
y_pred_bi = label_binarize(y_pred, classes=[0,1,2,3,4,5,6,7,8,9,10,11,12,13,14,15,16,17,18, 19,20,21,2,23,24,25,26,27,28,29,30,31,32,33,34,35,36,37,38,3,40,41,42,43,44,45])
# Compute ROC curve and ROC area for each class
fpr = dict()
tpr = dict()
roc_auc = dict()
for i in range(2):
fpr[i], tpr[i], _ = roc_curve(y_test_bi, y_pred_bi)
roc_auc[i] = auc(fpr[i], tpr[i])
However, now I'm getting the following error:
Traceback (most recent call last):
File "C:\Users\app\Documents\Python Scripts\gbc_classifier_test.py", line 152, in <module>
fpr[i], tpr[i], _ = roc_curve(y_test_bi, y_pred_bi)
File "C:\Users\app\Anaconda\lib\site-packages\sklearn\metrics\metrics.py", line 672, in roc_curve
fps, tps, thresholds = _binary_clf_curve(y_true, y_score, pos_label)
File "C:\Users\app\Anaconda\lib\site-packages\sklearn\metrics\metrics.py", line 505, in _binary_clf_curve
y_true = column_or_1d(y_true)
File "C:\Users\app\Anaconda\lib\site-packages\sklearn\utils\validation.py", line 265, in column_or_1d
raise ValueError("bad input shape {0}".format(shape))
ValueError: bad input shape (300L, 46L)
roc_curve
takes parameter with shape [n_samples]
(link), and your inputs (either y_test_bi
or y_pred_bi
) are of shape (300, 46)
. Note the first
I think the problem is y_pred_bi
is an array of probabilities, created by calling clf.predict_proba(X)
(please confirm this). Since your classifier was trained on all 46 classes, it outputs a 46-dimensional vectors for each data point, and there is nothing label_binarize
can do about that.
I know of two ways around this:
- Train 46 binary classifiers by invoking
label_binarize
before clf.fit()
and then compute ROC curve
- Slice each column of the 300-by-46 output array and pass that as the second parameter to
roc_curve
. This is my preferred approach by I am assuming y_pred_bi
contains probabilities
Use label_binarize
:
import matplotlib.pyplot as plt
from sklearn import svm, datasets
from sklearn.model_selection import train_test_split
from sklearn.preprocessing import label_binarize
from sklearn.metrics import roc_curve, auc
from sklearn.multiclass import OneVsRestClassifier
iris = datasets.load_iris()
X = iris.data
y = iris.target
# Binarize the output
y = label_binarize(y, classes=[0, 1, 2])
n_classes = y.shape[1]
X_train, X_test, y_train, y_test = train_test_split(X, y, test_size=.5, random_state=0)
classifier = OneVsRestClassifier(svm.SVC(kernel='linear', probability=True,
random_state=0))
y_score = classifier.fit(X_train, y_train).decision_function(X_test)
fpr = dict()
tpr = dict()
roc_auc = dict()
for i in range(n_classes):
fpr[i], tpr[i], _ = roc_curve(y_test[:, i], y_score[:, i])
roc_auc[i] = auc(fpr[i], tpr[i])
colors = cycle(['blue', 'red', 'green'])
for i, color in zip(range(n_classes), colors):
plt.plot(fpr[i], tpr[i], color=color, lw=lw,
label='ROC curve of class {0} (area = {1:0.2f})'
''.format(i, roc_auc[i]))
plt.plot([0, 1], [0, 1], 'k--', lw=lw)
plt.xlim([-0.05, 1.0])
plt.ylim([0.0, 1.05])
plt.xlabel('False Positive Rate')
plt.ylabel('True Positive Rate')
plt.title('Receiver operating characteristic for multi-class data')
plt.legend(loc="lower right")
plt.show()
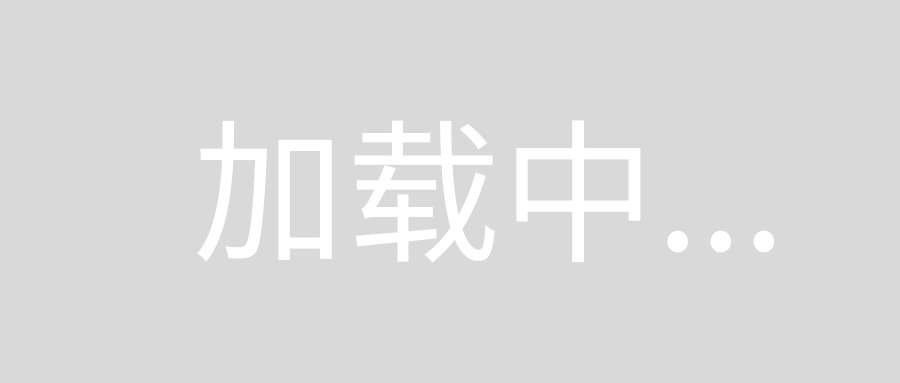