We would like to move data from DynamoDB NoSQL into Redshift Database continously as a stream.
I am having hard time understand all the new terms/technologies in AWS. There is
1) DynamoDB Streams
2) AWS Lambda
3) AWS Kinesis Firehose
Can someone provide a brief summary of each.
What are DynamoDB streams?
How does this differ from AmazonKinesis?
After reading all the resources, this is my hypothesis understanding, please verify below.
(a) I assume DynamoDB Streams, create the streaming data of NoSQL, and start sending it out. It is the Sender.
(b) Lambda allows people for only time consumed, it is the time for rent Server which handles the DynamoDB Stream.
(c) Kinesis FireHose Converts the DynamoDB Stream, and places into Redshift.
(d) AmazonQuickSight is their business intelligence tool,
Is that the correct understanding of the glossary terms?
Reviewing Stack link, wanted more thorough information.
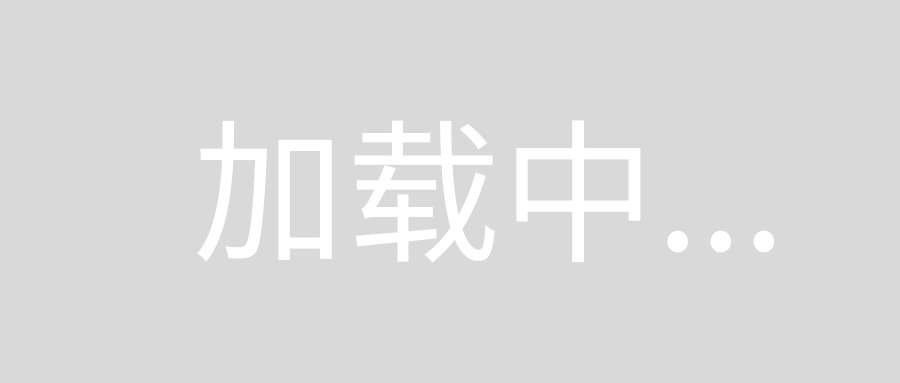
Amazon Kinesis can collect, process, and analyze video and data streams in real time.
- Use Kinesis Video Streams to capture, process, and store video streams for analytics and machine learning.
- Use Kinesis Data Streams to build custom applications that analyze data streams using popular stream processing frameworks.
- Use Kinesis Data Firehose to load data streams into AWS data stores.
- Use Kinesis Data Analytics to analyze data streams with SQL.
DynamoDB streams are effective the same as a Kinesis Data Stream, but it is automatically generated by new/changed data in DynamoDB. This allows applications to be notified when new data is added to a DynamoDB table, or when data is changed.
A Kinesis Data Firehose can automatically output a stream into Redshift (amongst other destinations).
AWS Lambda can run code without provisioning or managing servers. You pay only for the compute time you consume — there's no charge when your code isn't running. You can run code for virtually any type of application or backend service — all with zero administration.
Lambda is useful for inspecting data coming through a stream. For example, it could be used to manipulate the data format or skip-over data that is not required.
Putting it all together, you could have data added/modified in DynamoDB. This would cause a DynamoDB Stream to be sent that contains information about the change. An AWS Lambda function could inspect the data and manipulate/drop the message. If could then forward the data to Kinesis Data Firehose to automatically insert the data into Amazon Redshift.
Here's an example:
- A bank transaction is stored in DynamoDB
- DynamoDB Streams sends it to a Lambda function
- The Lambda function looks at the transaction and also retrieves information about the bank account. If there is sufficient balance in the account, the function exits and does nothing.
- If there is insufficient balance in the account, it could send an email via Amazon SES telling the account holder. It could then send the data to Firehose that stores it in Redshift for reporting of overdue accounts.
The benefit of using these systems together is that they can provide rich application functionality with minimal coding. In this example, only the Lambda function needed coding -- the rest worked via linking together various components. Also, it was totally serverless — that is, there was no need to run an application on an Amazon EC2 instance.