I want to make a custom layer which is supposed to fuse the output of a Dense Layer with a Convolution2D Layer.
The Idea came from this paper and here's the network:
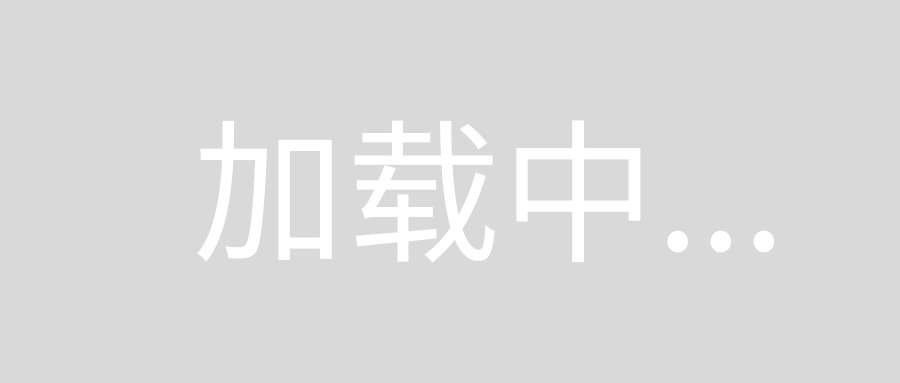
the fusion layer tries to fuse the Convolution2D tensor (256x28x28
) with the Dense tensor (256
). here's the equation for it:
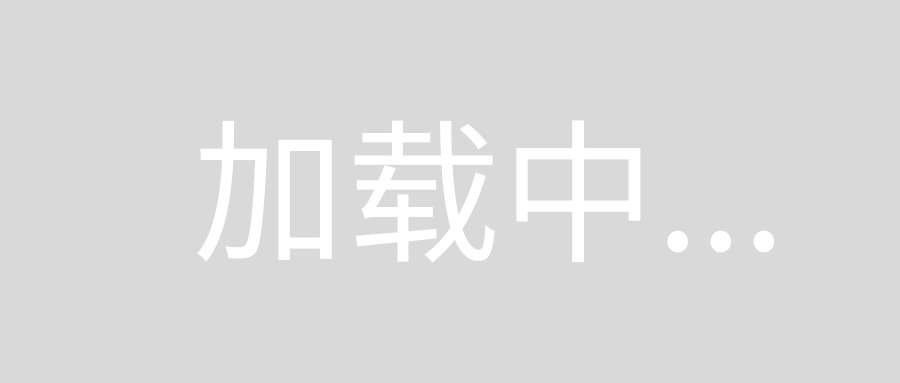
y_global => Dense layer output with shape 256
y_mid => Convolution2D layer output with shape 256x28x28
Here's the description of the paper about the Fusion process:
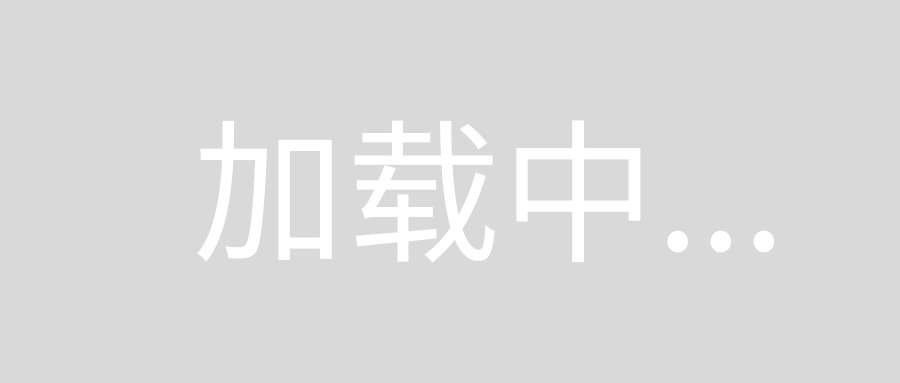
I ended up making a new custom layer like below:
class FusionLayer(Layer):
def __init__(self, output_dim, **kwargs):
self.output_dim = output_dim
super(FusionLayer, self).__init__(**kwargs)
def build(self, input_shape):
input_dim = input_shape[1][1]
initial_weight_value = np.random.random((input_dim, self.output_dim))
self.W = K.variable(initial_weight_value)
self.b = K.zeros((input_dim,))
self.trainable_weights = [self.W, self.b]
def call(self, inputs, mask=None):
y_global = inputs[0]
y_mid = inputs[1]
# the code below should be modified
output = K.dot(K.concatenate([y_global, y_mid]), self.W)
output += self.b
return self.activation(output)
def get_output_shape_for(self, input_shape):
assert input_shape and len(input_shape) == 2
return (input_shape[0], self.output_dim)
I think I got the __init__
and build
methods right but I don't know how to concatenate y_global
(256 dimesnions) with y-mid
(256x28x28 dimensions) in the call
layer so that the output would be the same as the equation mentioned above.
How can I implement this equation in the call
method?
Thanks so much...
UPDATE: any other way to successfully integrate the data of these 2 layers is also acceptable for me... it doesn't exactly have to be the way mentioned in the paper but it needs to at least return an acceptable output...
I had to ask this question on the Keras Github page and someone helped me on how to implement it properly... here's the issue on github...
I was working on a project of Image Colorization and ended up facing a fusion layer problem then I found a model containing fusion Layer.
Here it is Hope that solves your questions to some extent.
embed_input = Input(shape=(1000,))
encoder_input = Input(shape=(256, 256, 1,))
#Encoder
encoder_output = Conv2D(64, (3,3), activation='relu', padding='same', strides=2,
bias_initializer=TruncatedNormal(mean=0.0, stddev=0.05))(encoder_input)
encoder_output = Conv2D(128, (3,3), activation='relu', padding='same',
bias_initializer=TruncatedNormal(mean=0.0, stddev=0.05))(encoder_output)
encoder_output = Conv2D(128, (3,3), activation='relu', padding='same', strides=2,
bias_initializer=TruncatedNormal(mean=0.0, stddev=0.05))(encoder_output)
encoder_output = Conv2D(256, (3,3), activation='relu', padding='same',
bias_initializer=TruncatedNormal(mean=0.0, stddev=0.05))(encoder_output)
encoder_output = Conv2D(256, (3,3), activation='relu', padding='same', strides=2,
bias_initializer=TruncatedNormal(mean=0.0, stddev=0.05))(encoder_output)
encoder_output = Conv2D(512, (3,3), activation='relu', padding='same',
bias_initializer=TruncatedNormal(mean=0.0, stddev=0.05))(encoder_output)
encoder_output = Conv2D(512, (3,3), activation='relu', padding='same',
bias_initializer=TruncatedNormal(mean=0.0, stddev=0.05))(encoder_output)
encoder_output = Conv2D(256, (3,3), activation='relu', padding='same',
bias_initializer=TruncatedNormal(mean=0.0, stddev=0.05))(encoder_output)
#Fusion
fusion_output = RepeatVector(32 * 32)(embed_input)
fusion_output = Reshape(([32, 32, 1000]))(fusion_output)
fusion_output = concatenate([encoder_output, fusion_output], axis=3)
fusion_output = Conv2D(256, (1, 1), activation='relu', padding='same',
bias_initializer=TruncatedNormal(mean=0.0, stddev=0.05))(fusion_output)
#Decoder
decoder_output = Conv2D(128, (3,3), activation='relu', padding='same',
bias_initializer=TruncatedNormal(mean=0.0, stddev=0.05))(fusion_output)
decoder_output = UpSampling2D((2, 2))(decoder_output)
decoder_output = Conv2D(64, (3,3), activation='relu', padding='same',
bias_initializer=TruncatedNormal(mean=0.0, stddev=0.05))(decoder_output)
decoder_output = UpSampling2D((2, 2))(decoder_output)
decoder_output = Conv2D(32, (3,3), activation='relu', padding='same',
bias_initializer=TruncatedNormal(mean=0.0, stddev=0.05))(decoder_output)
decoder_output = Conv2D(16, (3,3), activation='relu', padding='same',
bias_initializer=TruncatedNormal(mean=0.0, stddev=0.05))(decoder_output)
decoder_output = Conv2D(2, (3, 3), activation='tanh', padding='same',
bias_initializer=TruncatedNormal(mean=0.0, stddev=0.05))(decoder_output)
decoder_output = UpSampling2D((2, 2))(decoder_output)
model = Model(inputs=[encoder_input, embed_input], outputs=decoder_output)
here is the source link: https://github.com/hvvashistha/Auto-Colorize
In my opinion implementing a new kind of layer is a way to complicated for this task. I strongly advise you to use the following layers:
in order to obtain the expected behaviour.