This article illustrates how to add Runtime statistics to Tensorboard:
run_options = tf.RunOptions(trace_level=tf.RunOptions.FULL_TRACE)
run_metadata = tf.RunMetadata()
summary, _ = sess.run([merged, train_step],
feed_dict=feed_dict(True),
options=run_options,
run_metadata=run_metadata)
train_writer.add_run_metadata(run_metadata, 'step%d' % i)
train_writer.add_summary(summary, i)
print('Adding run metadata for', i)
which creates the following details in Tensorboard:
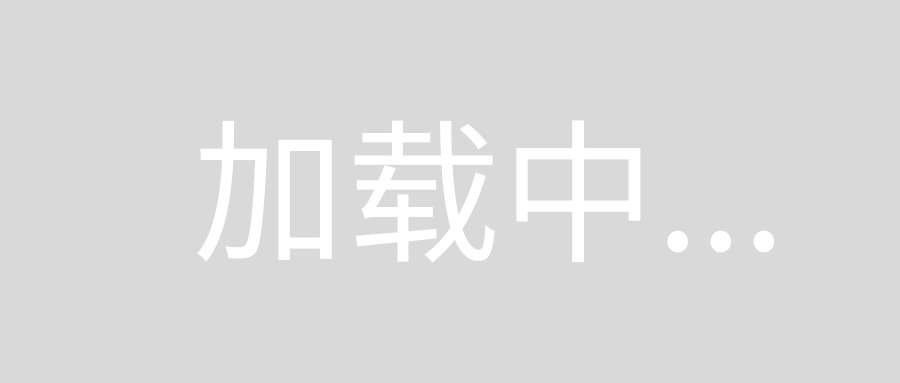
This is fairly straightforward on a single machine. How could one do this in a distributed environment using Estimators?
You may use tf.train.ProfilerHook. However the catch is that it was released at 1.14.
Example usage:
estimator = tf.estimator.LinearClassifier(...)
hooks = [tf.train.ProfilerHook(output_dir=model_dir, save_secs=600, show_memory=False)]
estimator.train(input_fn=train_input_fn, hooks=hooks)
Executing the hook will generate files timeline-xx.json
in output_dir
.
Then open chrome://tracing/
in chrome browser and load the file. You will get a time usage timeline like below.
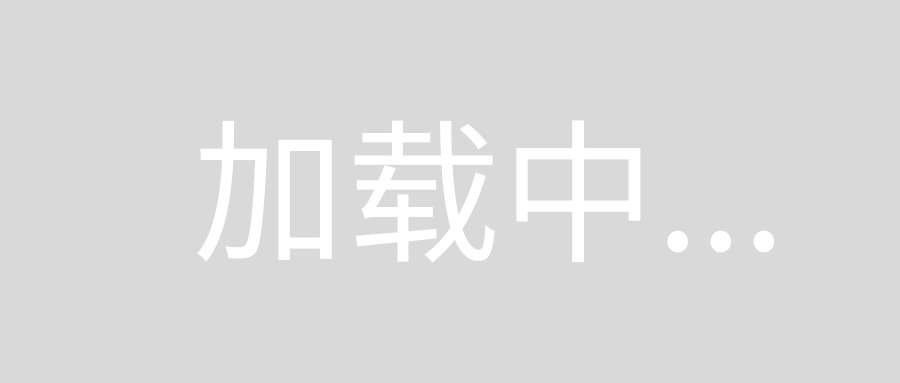
I use the following hook, based on ProfilerHook, to have the estimator output the run metadata into the model directory and inspect it later with Tensorboard.
import tensorflow as tf
from tensorflow.python.training.session_run_hook import SessionRunHook, SessionRunArgs
from tensorflow.python.training import training_util
from tensorflow.python.training.basic_session_run_hooks import SecondOrStepTimer
class MetadataHook(SessionRunHook):
def __init__ (self,
save_steps=None,
save_secs=None,
output_dir=""):
self._output_tag = "step-{}"
self._output_dir = output_dir
self._timer = SecondOrStepTimer(
every_secs=save_secs, every_steps=save_steps)
def begin(self):
self._next_step = None
self._global_step_tensor = training_util.get_global_step()
self._writer = tf.summary.FileWriter (self._output_dir, tf.get_default_graph())
if self._global_step_tensor is None:
raise RuntimeError("Global step should be created to use ProfilerHook.")
def before_run(self, run_context):
self._request_summary = (
self._next_step is None or
self._timer.should_trigger_for_step(self._next_step)
)
requests = {"global_step": self._global_step_tensor}
opts = (tf.RunOptions(trace_level=tf.RunOptions.FULL_TRACE)
if self._request_summary else None)
return SessionRunArgs(requests, options=opts)
def after_run(self, run_context, run_values):
stale_global_step = run_values.results["global_step"]
global_step = stale_global_step + 1
if self._request_summary:
global_step = run_context.session.run(self._global_step_tensor)
self._writer.add_run_metadata(
run_values.run_metadata, self._output_tag.format(global_step))
self._writer.flush()
self._next_step = global_step + 1
def end(self, session):
self._writer.close()
To use it, one creates the estimator instance (my_estimator) as usual, whether it is pre-made one or a custom estimator. The desired operation is called passing an instance of the class above as a hook. For example:
hook = MetadataHook(save_steps=1, output_dir=<model dir>)
my_estimator.train( train_input_fn, hooks=[hook] )
The run metadata will be placed in the model dir and can be inspected by TensorBoard.