I need to rbind two large data frames. Right now I use
df <- rbind(df, df.extension)
but I (almost) instantly run out of memory. I guess its because df is held in the memory twice. I might see even bigger data frames in the future, so I need some kind of in-place rbind.
So my question is: Is there a way to avoid data duplication in memory when using rbind?
I found this question, which uses SqlLite, but I really want to avoid using the hard drive as a cache.
data.table
is your friend!
C.f. http://www.mail-archive.com/r-help@r-project.org/msg175877.html
Following up on nikola's comment, here is ?rbindlist
's description (new in v1.8.2) :
Same as do.call("rbind",l)
, but much faster.
First of all : Use the solution from the other question you link to if you want to be safe. As R is call-by-value, forget about an "in-place" method that doesn't copy your dataframes in the memory.
One not advisable method of saving quite a bit of memory, is to pretend your dataframes are lists, coercing a list using a for-loop (apply will eat memory like hell) and make R believe it actually is a dataframe.
I'll warn you again : using this on more complex dataframes is asking for trouble and hard-to-find bugs. So be sure you test well enough, and if possible, avoid this as much as possible.
You could try following approach :
n1 <- 1000000
n2 <- 1000000
ncols <- 20
dtf1 <- as.data.frame(matrix(sample(n1*ncols), n1, ncols))
dtf2 <- as.data.frame(matrix(sample(n2*ncols), n1, ncols))
dtf <- list()
for(i in names(dtf1)){
dtf[[i]] <- c(dtf1[[i]],dtf2[[i]])
}
attr(dtf,"row.names") <- 1:(n1+n2)
attr(dtf,"class") <- "data.frame"
It erases rownames you actually had (you can reconstruct them, but check for duplicate rownames!). It also doesn't carry out all the other tests included in rbind.
Saves you about half of the memory in my tests, and in my test both the dtfcomb and the dtf are equal. The red box is rbind, the yellow one is my list-based approach.
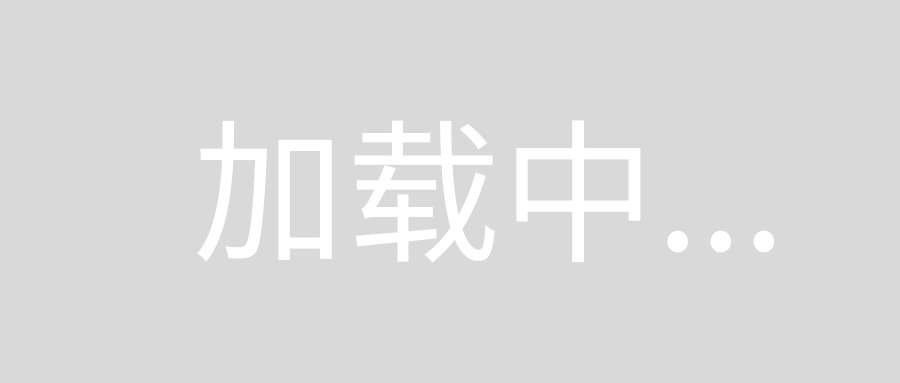
Test script :
n1 <- 3000000
n2 <- 3000000
ncols <- 20
dtf1 <- as.data.frame(matrix(sample(n1*ncols), n1, ncols))
dtf2 <- as.data.frame(matrix(sample(n2*ncols), n1, ncols))
gc()
Sys.sleep(10)
dtfcomb <- rbind(dtf1,dtf2)
Sys.sleep(10)
gc()
Sys.sleep(10)
rm(dtfcomb)
gc()
Sys.sleep(10)
dtf <- list()
for(i in names(dtf1)){
dtf[[i]] <- c(dtf1[[i]],dtf2[[i]])
}
attr(dtf,"row.names") <- 1:(n1+n2)
attr(dtf,"class") <- "data.frame"
Sys.sleep(10)
gc()
Sys.sleep(10)
rm(dtf)
gc()
Right now I worked out the following solution:
nextrow = nrow(df)+1
df[nextrow:(nextrow+nrow(df.extension)-1),] = df.extension
# we need to assure unique row names
row.names(df) = 1:nrow(df)
Now I don't run out of memory. I think its because I store
object.size(df) + 2 * object.size(df.extension)
while with rbind R would need
object.size(rbind(df,df.extension)) + object.size(df) + object.size(df.extension).
After that I use
rm(df.extension)
gc(reset=TRUE)
to free the memory I don't need anymore.
This solved my problem for now, but I feel that there is a more advanced way to do a memory efficient rbind. I appreciate any comments on this solution.
This is a perfect candidate for bigmemory
. See the site for more information. Here are three usage aspects to consider:
- It's OK to use the HD: Memory mapping to the HD is much faster than practically any other access, so you may not see any slowdowns. At times I rely upon > 1TB of memory-mapped matrices, though most are between 6 and 50GB. Moreover, as the object is a matrix, this requires no real overhead of rewriting code in order to use the object.
- Whether you use a file-backed matrix or not, you can use
separated = TRUE
to make the columns separate. I haven't used this much, because of my 3rd tip:
- You can over-allocate the HD space to allow for a larger potential matrix size, but only load the submatrix of interest. This way there is no need to do
rbind
.
Note: Although the original question addressed data frames and bigmemory is suitable for matrices, one can easily create different matrices for different types of data and then combine the objects in RAM to create a dataframe, if it's really necessary.