I'm looking at the documentation, and here is their example. I cannot understand how this example corresponds to their explanation: "Returns a new tensor with a dimension of size one inserted at the specified position."
>>> x = torch.tensor([1, 2, 3, 4])
>>> torch.unsqueeze(x, 0)
tensor([[ 1, 2, 3, 4]])
>>> torch.unsqueeze(x, 1)
tensor([[ 1],
[ 2],
[ 3],
[ 4]])
If you look at the shape of the array before and after, you see that before it was (4,)
and after it is (1, 4)
(when second parameter is 0
) and (4, 1)
(when second parameter is 1
). So a 1
was inserted in the shape of the array at axis 0
or 1
, depending on the value of the second parameter.
That is opposite of np.squeeze()
(nomenclature borrowed from MATLAB) which removes axes of size 1
(singletons).
I am not sure why PyTorch references are not mentioned here since this is PyTorch legacy.
torch.squeeze
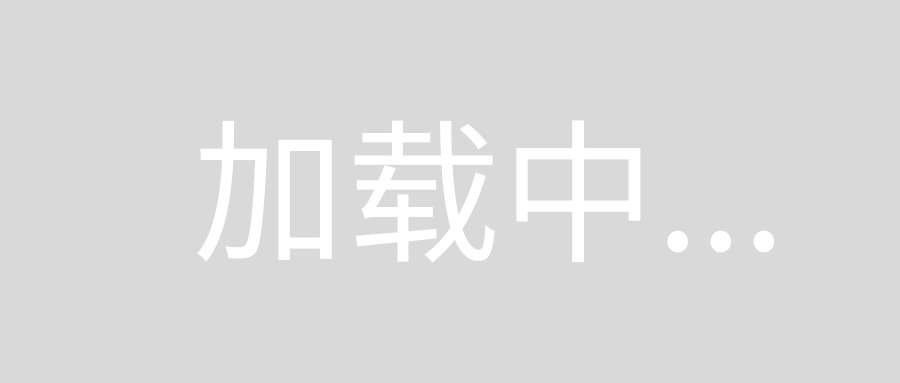
torch.unsqueeze
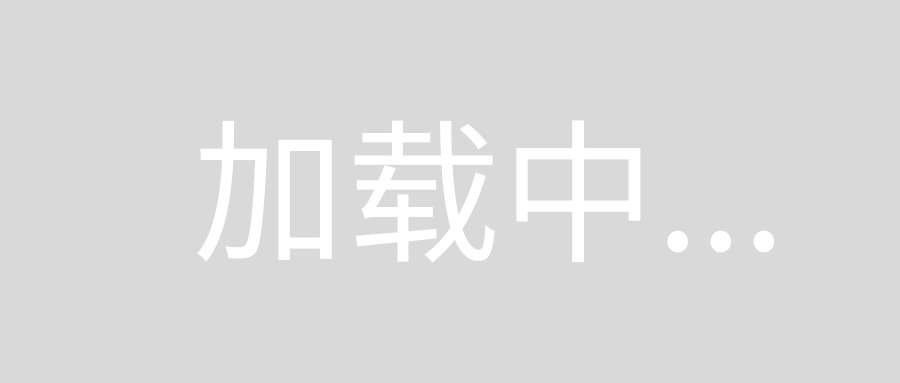
It indicates the position on where to add the dimension. torch.unsqueeze adds an additional dimension to the tensor. So let's say you have a tensor of shape (3), if you add a dimension at the 0 position, it will be of shape (1,3), which means 1 row and 3 columns. If you add at the 1 position, it will be (3,1), which means 3 rows and 1 column. If you have a 2D tensor of shape (2,2) add add an extra dimension at the 0 position, this will result of the tensor having a shape of (1,2,2), which means one channel, 2 rows and 2 columns. If you add at the 1 position, it will be of shape (2,1,2), so it will have 2 channels, 1 row and 2 columns. If you add it at the 2 position, the tensor will be of shape (2,2,1), which means 2 channels, 2 rows and one column.