I would like find the fastes way in R to indentify indexes of elements in Ytimes array which are closest to given Xtimes values.
So far I have been using a simple for-loop, but there must be a better way to do it:
Xtimes <- c(1,5,8,10,15,19,23,34,45,51,55,57,78,120)
Ytimes <- seq(0,120,length.out = 1000)
YmatchIndex = array(0,length(Xtimes))
for (i in 1:length(Xtimes)) {
YmatchIndex[i] = which.min(abs(Ytimes - Xtimes[i]))
}
print(Ytimes[YmatchIndex])
R
is vectorized, so skip the for
loop. This saves time in scripting and computation. Simply replace the for
loop with an apply
function. Since we're returning a 1D vector, we use sapply
.
YmatchIndex <- sapply(Xtimes, function(x){which.min(abs(Ytimes - x))})
Proof that apply
is faster:
library(microbenchmark)
library(ggplot2)
# set up data
Xtimes <- c(1,5,8,10,15,19,23,34,45,51,55,57,78,120)
Ytimes <- seq(0,120,length.out = 1000)
# time it
mbm <- microbenchmark(
for_loop = for (i in 1:length(Xtimes)) {
YmatchIndex[i] = which.min(abs(Ytimes - Xtimes[i]))
},
apply = sapply(Xtimes, function(x){which.min(abs(Ytimes - x))}),
times = 100
)
# plot
autoplot(mbm)
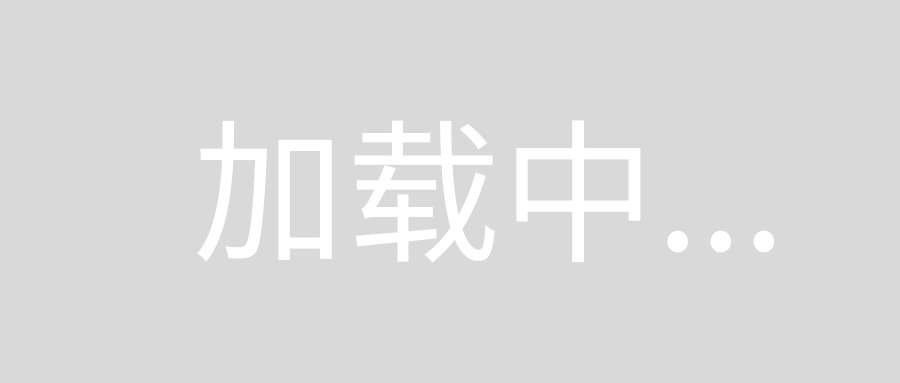
See ?apply for more
.
Obligatory Rcpp solution. Takes advantage of the fact that your vectors are sorted and don't contain duplicates to turn an O(n^2)
into an O(n)
. May or may not be practical for your application ;)
C++:
#include <Rcpp.h>
#include <cmath>
using namespace Rcpp;
// [[Rcpp::export]]
IntegerVector closest_pts(NumericVector Xtimes, NumericVector Ytimes) {
int xsize = Xtimes.size();
int ysize = Ytimes.size();
int y_ind = 0;
double minval = R_PosInf;
IntegerVector output(xsize);
for(int x_ind = 0; x_ind < xsize; x_ind++) {
while(std::abs(Ytimes[y_ind] - Xtimes[x_ind]) < minval) {
minval = std::abs(Ytimes[y_ind] - Xtimes[x_ind]);
y_ind++;
}
output[x_ind] = y_ind;
minval = R_PosInf;
}
return output;
}
R:
microbenchmark::microbenchmark(
for_loop = {
for (i in 1:length(Xtimes)) {
which.min(abs(Ytimes - Xtimes[i]))
}
},
apply = sapply(Xtimes, function(x){which.min(abs(Ytimes - x))}),
fndIntvl = {
Y2 <- c(-Inf, Ytimes + c(diff(Ytimes)/2, Inf))
Ytimes[ findInterval(Xtimes, Y2) ]
},
rcpp = closest_pts(Xtimes, Ytimes),
times = 100
)
Unit: microseconds
expr min lq mean median uq max neval cld
for_loop 3321.840 3422.51 3584.452 3492.308 3624.748 10458.52 100 b
apply 68.365 73.04 106.909 84.406 93.097 2345.26 100 a
fndIntvl 31.623 37.09 50.168 42.019 64.595 105.14 100 a
rcpp 2.431 3.37 5.647 4.301 8.259 10.76 100 a
identical(closest_pts(Xtimes, Ytimes), findInterval(Xtimes, Y2))
# TRUE
We can use findInterval
to do this efficiently. (cut
will also work, with a little more work).
First, let's offset the Ytimes
offsets so that we can find the nearest and not the next-lesser. I'll demonstrate on fake data first:
y <- c(1,3,5,10,20)
y2 <- c(-Inf, y + c(diff(y)/2, Inf))
cbind(y, y2[-1])
# y
# [1,] 1 2.0
# [2,] 3 4.0
# [3,] 5 7.5
# [4,] 10 15.0
# [5,] 20 Inf
findInterval(c(1, 1.9, 2.1, 8), y2)
# [1] 1 1 2 4
The second column (prepended with a -Inf
will give us the breaks. Notice that each is half-way between the corresponding value and its follower.
Okay, let's apply this to your vectors:
Y2 <- Ytimes + c(diff(Ytimes)/2, Inf)
head(cbind(Ytimes, Y2))
# Ytimes Y2
# [1,] 0.0000000 0.06006006
# [2,] 0.1201201 0.18018018
# [3,] 0.2402402 0.30030030
# [4,] 0.3603604 0.42042042
# [5,] 0.4804805 0.54054054
# [6,] 0.6006006 0.66066066
Y2 <- c(-Inf, Ytimes + c(diff(Ytimes)/2, Inf))
cbind(Xtimes, Y2[ findInterval(Xtimes, Y2) ])
# Xtimes
# [1,] 1 0.9009009
# [2,] 5 4.9849850
# [3,] 8 7.9879880
# [4,] 10 9.9099099
# [5,] 15 14.9549550
# [6,] 19 18.9189189
# [7,] 23 22.8828829
# [8,] 34 33.9339339
# [9,] 45 44.9849850
# [10,] 51 50.9909910
# [11,] 55 54.9549550
# [12,] 57 56.9969970
# [13,] 78 77.8978979
# [14,] 120 119.9399399
(I'm using cbind
just for side-by-side demonstration, not that it's necessary.)
Benchmark:
mbm <- microbenchmark::microbenchmark(
for_loop = {
YmatchIndex <- array(0,length(Xtimes))
for (i in 1:length(Xtimes)) {
YmatchIndex[i] = which.min(abs(Ytimes - Xtimes[i]))
}
},
apply = sapply(Xtimes, function(x){which.min(abs(Ytimes - x))}),
fndIntvl = {
Y2 <- c(-Inf, Ytimes + c(diff(Ytimes)/2, Inf))
Ytimes[ findInterval(Xtimes, Y2) ]
},
times = 100
)
mbm
# Unit: microseconds
# expr min lq mean median uq max neval
# for_loop 2210.5 2346.8 2823.678 2444.80 3029.45 7800.7 100
# apply 48.8 58.7 100.455 65.55 91.50 2568.7 100
# fndIntvl 18.3 23.4 34.059 29.80 40.30 83.4 100
ggplot2::autoplot(mbm)
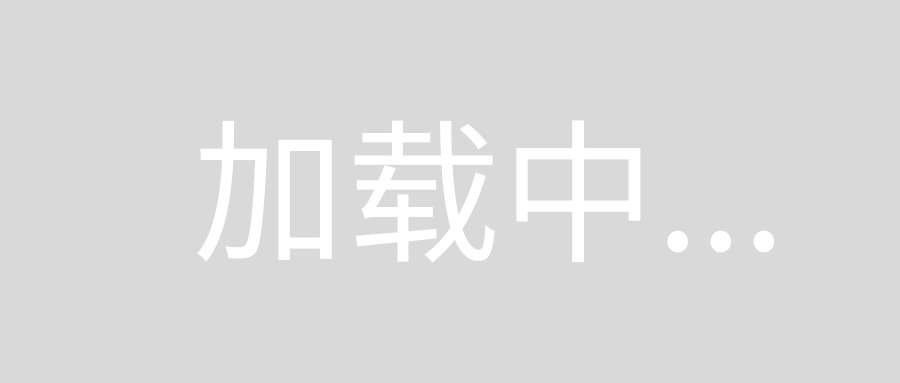