可以将文章内容翻译成中文,广告屏蔽插件可能会导致该功能失效(如失效,请关闭广告屏蔽插件后再试):
问题:
import pandas as pd
import numpy as np
import cv2
from torch.utils.data.dataset import Dataset
class CustomDatasetFromCSV(Dataset):
def __init__(self, csv_path, transform=None):
self.data = pd.read_csv(csv_path)
self.labels = pd.get_dummies(self.data['emotion']).as_matrix()
self.height = 48
self.width = 48
self.transform = transform
def __getitem__(self, index):
pixels = self.data['pixels'].tolist()
faces = []
for pixel_sequence in pixels:
face = [int(pixel) for pixel in pixel_sequence.split(' ')]
# print(np.asarray(face).shape)
face = np.asarray(face).reshape(self.width, self.height)
face = cv2.resize(face.astype('uint8'), (self.width, self.height))
faces.append(face.astype('float32'))
faces = np.asarray(faces)
faces = np.expand_dims(faces, -1)
return faces, self.labels
def __len__(self):
return len(self.data)
This is what I could manage to do by using references from other repositories.
However, I want to split this dataset into train and test.
How can I do that inside this class? Or do I need to make a separate class to do that?
回答1:
Using Pytorch's SubsetRandomSampler
:
import torch
import numpy as np
from torchvision import datasets
from torchvision import transforms
from torch.utils.data.sampler import SubsetRandomSampler
class CustomDatasetFromCSV(Dataset):
def __init__(self, csv_path, transform=None):
self.data = pd.read_csv(csv_path)
self.labels = pd.get_dummies(self.data['emotion']).as_matrix()
self.height = 48
self.width = 48
self.transform = transform
def __getitem__(self, index):
# This method should return only 1 sample and label
# (according to "index"), not the whole dataset
# So probably something like this for you:
pixel_sequence = self.data['pixels'][index]
face = [int(pixel) for pixel in pixel_sequence.split(' ')]
face = np.asarray(face).reshape(self.width, self.height)
face = cv2.resize(face.astype('uint8'), (self.width, self.height))
label = self.labels[index]
return face, label
def __len__(self):
return len(self.labels)
dataset = CustomDatasetFromCSV(my_path)
batch_size = 16
validation_split = .2
shuffle_dataset = True
random_seed= 42
# Creating data indices for training and validation splits:
dataset_size = len(dataset)
indices = list(range(dataset_size))
split = int(np.floor(validation_split * dataset_size))
if shuffle_dataset :
np.random.seed(random_seed)
np.random.shuffle(indices)
train_indices, val_indices = indices[split:], indices[:split]
# Creating PT data samplers and loaders:
train_sampler = SubsetRandomSampler(train_indices)
valid_sampler = SubsetRandomSampler(val_indices)
train_loader = torch.utils.data.DataLoader(dataset, batch_size=batch_size,
sampler=train_sampler)
validation_loader = torch.utils.data.DataLoader(dataset, batch_size=batch_size,
sampler=valid_sampler)
# Usage Example:
num_epochs = 10
for epoch in range(num_epochs):
# Train:
for batch_index, (faces, labels) in enumerate(train_loader):
# ...
回答2:
Starting in PyTorch 0.4.1 you can use random_split
:
train_size = int(0.8 * len(full_dataset))
test_size = len(full_dataset) - train_size
train_dataset, test_dataset = torch.utils.data.random_split(full_dataset, [train_size, test_size])
回答3:
Current answers do random splits which has disadvantage that number of samples per class is not guaranteed to be balanced. This is especially problematic when you want to have small number of samples per class. For example, MNIST has 60,000 examples, i.e. 6000 per digit. Assume that you want only 30 examples per digit in your training set. In this case, random split may produce imbalance between classes (one digit with more training data then others). So you want to make sure each digit precisely has only 30 labels. This is called stratified sampling.
One way to do this is using sampler interface in Pytorch and sample code is here.
Another way to do this is just hack your way through :). For example, below is simple implementation for MNIST where ds
is MNIST dataset and k
is number of samples needed for each class.
def sampleFromClass(ds, k):
class_counts = {}
train_data = []
train_label = []
test_data = []
test_label = []
for data, label in ds:
c = label.item()
class_counts[c] = class_counts.get(c, 0) + 1
if class_counts[c] <= k:
train_data.append(data)
train_label.append(torch.unsqueeze(label, 0))
else:
test_data.append(data)
test_label.append(torch.unsqueeze(label, 0))
train_data = torch.cat(train_data)
for ll in train_label:
print(ll)
train_label = torch.cat(train_label)
test_data = torch.cat(test_data)
test_label = torch.cat(test_label)
return (TensorDataset(train_data, train_label),
TensorDataset(test_data, test_label))
You can use this function like this:
def main():
train_ds = datasets.MNIST('../data', train=True, download=True,
transform=transforms.Compose([
transforms.ToTensor()
]))
train_ds, test_ds = sampleFromClass(train_ds, 3)
回答4:
This is the PyTorch Subset
class attached holding the random_split
method. Note that this method is base for the SubsetRandomSampler
.
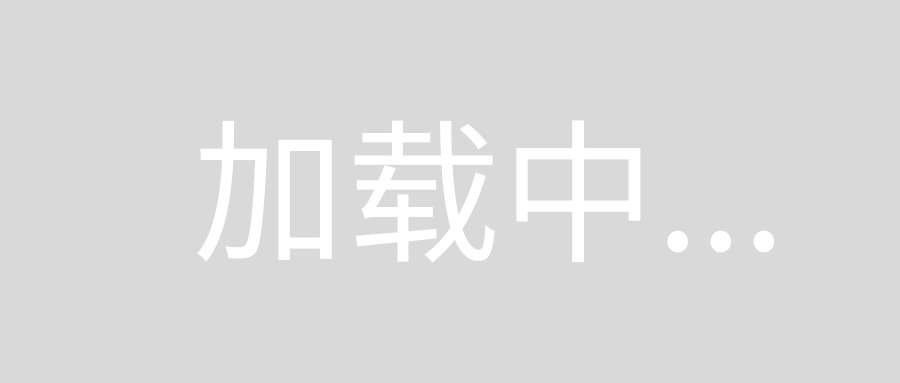
For MNIST if we use random_split
:
loader = DataLoader(
torchvision.datasets.MNIST('/data/mnist', train=True, download=True,
transform=torchvision.transforms.Compose([
torchvision.transforms.ToTensor(),
torchvision.transforms.Normalize(
(0.5,), (0.5,))
])),
batch_size=16, shuffle=False)
print(loader.dataset.data.shape)
test_ds, valid_ds = torch.utils.data.random_split(loader.dataset, (50000, 10000))
print(test_ds, valid_ds)
print(test_ds.indices, valid_ds.indices)
print(test_ds.indices.shape, valid_ds.indices.shape)
We get:
torch.Size([60000, 28, 28])
<torch.utils.data.dataset.Subset object at 0x0000020FD1880B00> <torch.utils.data.dataset.Subset object at 0x0000020FD1880C50>
tensor([ 1520, 4155, 45472, ..., 37969, 45782, 34080]) tensor([ 9133, 51600, 22067, ..., 3950, 37306, 31400])
torch.Size([50000]) torch.Size([10000])
Our test_ds.indices
and valid_ds.indices
will be random from range (0, 600000)
. But if I would like to get sequence of indices from (0, 49999)
and from (50000, 59999)
I cannot do that at the moment unfortunately, except this way.
Handy in case you run the MNIST benchmark where it is predefined what should be the test and what should be the validation dataset.
回答5:
Custom dataset has a special meaning in PyTorch, but I think you meant any dataset.
Let's check out the MNIST dataset (this is probable the most famous dataset for the beginners).
import torch, torchvision
import torchvision.datasets as datasets
from torch.utils.data import DataLoader, Dataset, TensorDataset
train_loader = DataLoader(
torchvision.datasets.MNIST('/data/mnist', train=True, download=True,
transform=torchvision.transforms.Compose([
torchvision.transforms.ToTensor(),
torchvision.transforms.Normalize(
(0.5,), (0.5,))
])),
batch_size=16, shuffle=False)
print(train_loader.dataset.data.shape)
test_ds = train_loader.dataset.data[:50000, :, :]
valid_ds = train_loader.dataset.data[50000:, :, :]
print(test_ds.shape)
print(valid_ds.shape)
test_dst = train_loader.dataset.targets.data[:50000]
valid_dst = train_loader.dataset.targets.data[50000:]
print(test_dst, test_dst.shape)
print(valid_dst, valid_dst.shape)
What this will outupt, is size of the original [60000, 28, 28]
, then the splits [50000, 28, 28]
for test and [10000, 28, 28]
for validation:
torch.Size([60000, 28, 28])
torch.Size([50000, 28, 28])
torch.Size([10000, 28, 28])
tensor([5, 0, 4, ..., 8, 4, 8]) torch.Size([50000])
tensor([3, 8, 6, ..., 5, 6, 8]) torch.Size([10000])
Additional info if you actually plan to pair images and labels (targets) together
bs = 16
test_dl = DataLoader(TensorDataset(test_ds, test_dst), batch_size=bs, shuffle=True)
for xb, yb in test_dl:
# Do your work