I have a dataframe which can be generated from the code as given below
df = pd.DataFrame({'person_id' :[1,2,3],'date1':
['12/31/2007','11/25/2009','10/06/2005'],'val1':
[2,4,6],'date2': ['12/31/2017','11/25/2019','10/06/2015'],'val2':[1,3,5],'date3':
['12/31/2027','11/25/2029','10/06/2025'],'val3':[7,9,11]})
I followed the below solution to convert it from wide to long
pd.wide_to_long(df, stubnames=['date', 'val'], i='person_id',
j='grp').sort_index(level=0)
Though this works with sample data as shown below, it doesn't work with my real data which has more than 200 columns. Instead of person_id, my real data has subject_ID which is values like DC0001,DC0002 etc. Does "I" always have to be numeric? Instead it adds the stub values as new columns in my dataset and has zero rows
This is how my real columns looks like
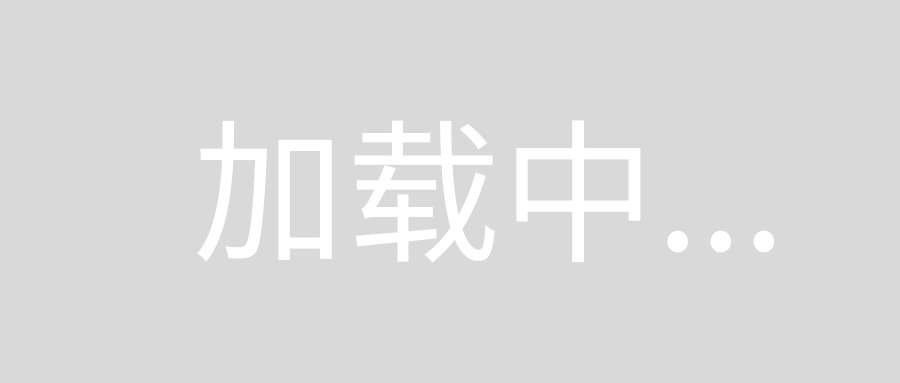
My real data might contains NA's as well. So do I have to fill them with default values for wide_to_long to work?
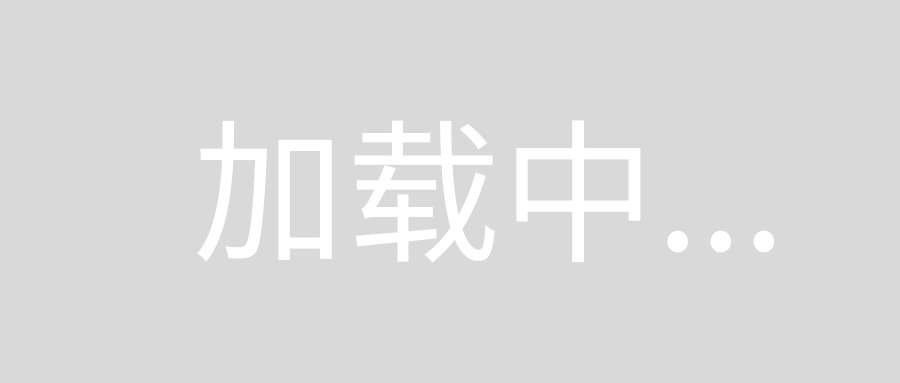
Can you please help as to what can be the issue? Or any other approach to achieve the same result is also helpful.
The issue is with your column names, the numbers used to convert from wide to long need to be at the end of your column names or you need to specify a suffix to groupby. I think the easiest solution is to create a function that accepts regex and the dataframe.
import pandas as pd
import re
def change_names(df, regex):
# Select one of three column groups
old_cols = df.filter(regex = regex).columns
# Create list of new column names
new_cols = []
for col in old_cols:
# Get the stubname of the original column
stub = ''.join(re.split(r'\d', col))
# Get the time point
num = re.findall(r'\d+', col) # returns a list like ['1']
# Make new column name
new_col = stub + num[0]
new_cols.append(new_col)
# Create dictionary mapping old column names to new column names
dd = {oc: nc for oc, nc in zip(old_cols, new_cols)}
# Rename columns
df.rename(columns = dd, inplace = True)
return df
tdf = pd.DataFrame({'person_id' :[1,2,3],'h1date': ['12/31/2007','11/25/2009','10/06/2005'],'t1val': [2,4,6],'h2date': ['12/31/2017','11/25/2019','10/06/2015'],'t2val':[1,3,5],'h3date': ['12/31/2027','11/25/2029','10/06/2025'],'t3val':[7,9,11]})
# Change date columns
tdf = change_names(tdf, 'date$')
tdf = change_names(tdf, 'val$')
print(tdf)
person_id hdate1 tval1 hdate2 tval2 hdate3 tval3
0 1 12/31/2007 2 12/31/2017 1 12/31/2027 7
1 2 11/25/2009 4 11/25/2019 3 11/25/2029 9
2 3 10/06/2005 6 10/06/2015 5 10/06/2025 11