I've been trying to superimpose a normal curve over my histogram with ggplot 2.
My formula:
data <- read.csv (path...)
ggplot(data, aes(V2)) +
geom_histogram(alpha=0.3, fill='white', colour='black', binwidth=.04)
I tried several things:
+ stat_function(fun=dnorm)
....didn't change anything
+ stat_density(geom = "line", colour = "red")
...gave me a straight red line on the x-axis.
+ geom_density()
doesn't work for me because I want to keep my frequency values on the y-axis, and want no density values.
Any suggestions?
Thanks in advance for any tips!
Solution found!
+geom_density(aes(y=0.045*..count..), colour="black", adjust=4)
This has been answered here and partially here.
If you want the y-axis to have frequency counts, then the normal curve needs to be scaled according to the number of observations and the binwidth.
# Simulate some data. Individuals' heights in cm.
n <- 1000
mean <- 165
sd <- 6.6
binwidth <- 2
height <- rnorm(n, mean, sd)
qplot(height, geom = "histogram", breaks = seq(130, 200, binwidth),
colour = I("black"), fill = I("white"),
xlab = "Height (cm)", ylab = "Count") +
# Create normal curve, adjusting for number of observations and binwidth
stat_function(
fun = function(x, mean, sd, n, bw){
dnorm(x = x, mean = mean, sd = sd) * n * bw
},
args = c(mean = mean, sd = sd, n = n, bw = binwidth))
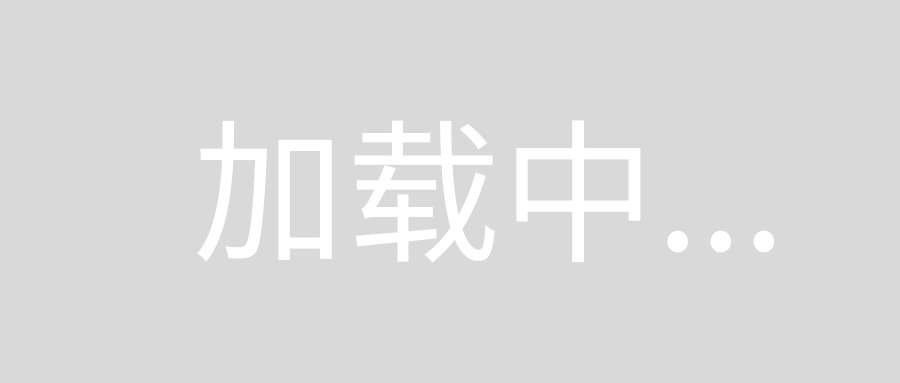
EDIT
Or, for a more flexible approach that allows for use of facets and draws upon an approach listed here, create a separate dataset containing the data for the normal curves and overlay these.
library(plyr)
dd <- data.frame(
predicted = rnorm(720, mean = 2, sd = 2),
state = rep(c("A", "B", "C"), each = 240)
)
binwidth <- 0.5
grid <- with(dd, seq(min(predicted), max(predicted), length = 100))
normaldens <- ddply(dd, "state", function(df) {
data.frame(
predicted = grid,
normal_curve = dnorm(grid, mean(df$predicted), sd(df$predicted)) * length(df$predicted) * binwidth
)
})
ggplot(dd, aes(predicted)) +
geom_histogram(breaks = seq(-3,10, binwidth), colour = "black", fill = "white") +
geom_line(aes(y = normal_curve), data = normaldens, colour = "red") +
facet_wrap(~ state)
Think I got it:
set.seed(1)
df <- data.frame(PF = 10*rnorm(1000))
ggplot(df, aes(x = PF)) +
geom_histogram(aes(y =..density..),
breaks = seq(-50, 50, by = 10),
colour = "black",
fill = "white") +
stat_function(fun = dnorm, args = list(mean = mean(df$PF), sd = sd(df$PF)))
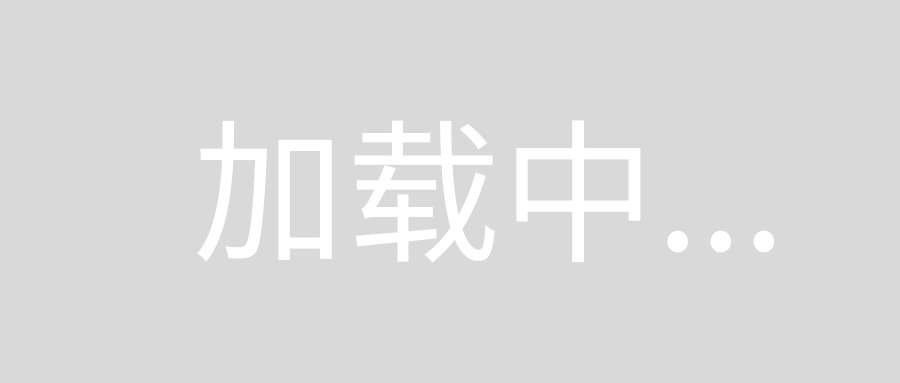
This is an extended comment on JWilliman's answer. I found J's answer very useful. While playing around I discovered a way to simplify the code. I'm not saying it is a better way, but I thought I would mention it.
Note that JWilliman's answer provides the count on the y-axis and a "hack" to scale the corresponding density normal approximation (which otherwise would cover a total area of 1 and have therefore a much lower peak).
Main point of this comment: simpler syntax inside stat_function
, by passing the needed parameters to the aesthetics function, e.g.
aes(x = x, mean = 0, sd = 1, binwidth = 0.3, n = 1000)
This avoids having to pass args =
to stat_function
and is therefore more user-friendly. Okay, it's not very different, but hopefully someone will find it interesting.
# parameters that will be passed to ``stat_function``
n = 1000
mean = 0
sd = 1
binwidth = 0.3 # passed to geom_histogram and stat_function
set.seed(1)
df <- data.frame(x = rnorm(n, mean, sd))
ggplot(df, aes(x = x, mean = mean, sd = sd, binwidth = binwidth, n = n)) +
theme_bw() +
geom_histogram(binwidth = binwidth,
colour = "white", fill = "cornflowerblue", size = 0.1) +
stat_function(fun = function(x) dnorm(x, mean = mean, sd = sd) * n * binwidth,
color = "darkred", size = 1)
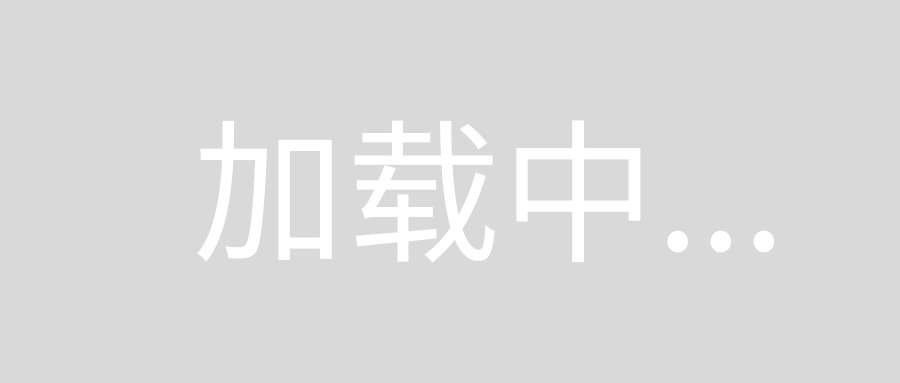
This code should do it:
set.seed(1)
z <- rnorm(1000)
qplot(z, geom = "blank") +
geom_histogram(aes(y = ..density..)) +
stat_density(geom = "line", aes(colour = "bla")) +
stat_function(fun = dnorm, aes(x = z, colour = "blabla")) +
scale_colour_manual(name = "", values = c("red", "green"),
breaks = c("bla", "blabla"),
labels = c("kernel_est", "norm_curv")) +
theme(legend.position = "bottom", legend.direction = "horizontal")
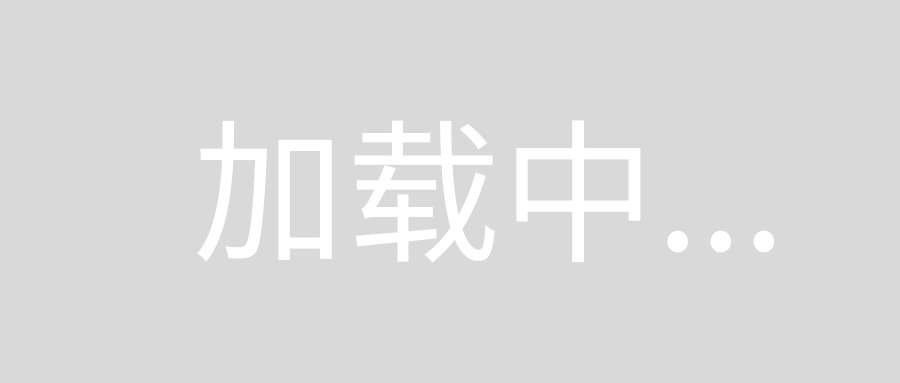
Note: I used qplot but you can use the more versatile ggplot.