我收集来自我的图像检测算法的结果。 所以基本上我做的是,从一组图像(具有320×480大小),我会跑的64x128滑动窗口通了,还下了一些预定义的尺度。
我明白那个:
- 真阳性=当我的检测窗口重叠(定义交集大小/质心内)与地面实况(注释边界框)
- 误报=当算法给我积极的窗口,这是grond真相之外。
- 假阴性=当它失败我给正窗口,而地面实况注解指出,有一个对象。
但对于真正的否定 ? 这些是真阴性所有的窗户,我的分类给我负的成绩? 这听起来很奇怪,因为我在同一时间通过滑动4个像素的小窗口(64x128),我已经应用于检测周围8个不同的尺度。 如果我要做到这一点,那么我有很多的每个图像真阴性。
或者,我准备了一套纯负面形象(无对象/人的话),在这里我只是滑动通,如果有一个在每个图像的一个或多个阳性检测,我就当漏判,副反之亦然?
下面是一个例子图像(绿色rects作为地面实况)
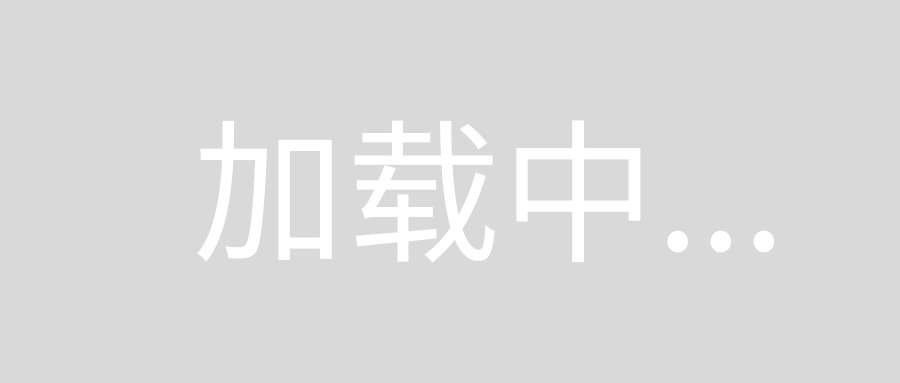
我始终认为,这四个方面,如下:
- 假阴性; 结果应该是积极的,但为负。
- 假阳性; 结果应该是阴性,但为正。
- 真阳性; 结果应该是积极的,是积极的。
- 真阴性; 结果应该是消极的和为负。
在你的情况,如果我理解正确的,你想,如果有在图像待检测对象。 因此假阴性就意味着有一个对象(结果应该是肯定的),但算法没有检测到它(并因此返回负)。 一个真正的负仅仅是算法正确地指出,其检查的区域不持有的对象。
您可以选择忽略负值,但这些可以被用来进一步训练你的算法(例如,使用一种算法,看起来而不是设置不被确认为假家居两者)。
AFAIK, 真阴性将是一个场景,其中一个对象是存在于图像中但仍未标记无论是在地面实况注解或模型预测。
通常2D物体探测系统只使用两个数据即地面真实注释和模型预测。 然而,要找到真正的负面情况下,我们需要寻求一个基本事实注解的超集,其中包含所有存在于图像(不只是那些具体到我们的模型)的类实例的信息。
例如进行给定的图像; 如果我们有兴趣做的自动驾驶目的,目标检测,我们可以考虑两个基本事实注解如下:
超集GT注解
- 汽车(车辆)
- 人
- 树
- 动物
- house_window
- 汉堡(也许扔在路上)
自动驾驶GT注解
随着上述两个基本事实注解将有可能来计算汉堡和窗口的真阴性。 但我怀疑,如果可以在不超注解来计算真阴性。
有一个很好的解释在这里 。 F1得分维基解释和这里是衡量成功很有帮助。
我试图写一个计算F1值的函数:
/// <param name="realClasses">Class names that exists on the image. A class name may exists more than once.</param>
/// <param name="foundClasses">Predicted class names. A class name may exists more than once.</param>
private static void findPosNeg(List<string> realClasses, List<string> foundClasses, out int truePositive, out int falsePositive, out int falseNegative)
{
Dictionary<string, int> dicReal = new Dictionary<string, int>(StringComparer.InvariantCultureIgnoreCase);
Dictionary<string, int> dicFound = new Dictionary<string, int>(StringComparer.InvariantCultureIgnoreCase);
#region fill dictionaries
foreach (string className in realClasses)
{
if (!dicReal.ContainsKey(className))
dicReal[className] = 1;
else
dicReal[className]++;
}
foreach (string className in foundClasses)
{
if (!dicFound.ContainsKey(className))
dicFound[className] = 1;
else
dicFound[className]++;
}
#endregion
truePositive = 0;
falsePositive = 0;
falseNegative = 0;
foreach (string className in dicFound.Keys)
{
if (!dicReal.ContainsKey(className))
falsePositive += dicFound[className];
else
{
int found = dicFound[className];
int real = dicReal[className];
truePositive += Math.Min(found, real);
if (real > found)
falseNegative += real - found;
else if (found > real)
falsePositive += found - real;
}
}
foreach (string className in dicReal.Keys)
if (!dicFound.ContainsKey(className))
falseNegative += dicReal[className];
}
/// <summary>
/// Calculates F1Score ref:https://en.wikipedia.org/wiki/Precision_and_recall
/// </summary>
private static double calc_F1Score(int truePositive, int falsePositive, int falseNegative, out double precision, out double recall)
{
precision = (double)truePositive / ((double)truePositive + (double)falsePositive);
recall = (double)truePositive / ((double)truePositive + (double)falseNegative);
double div = (precision + recall);
return (div != 0d) ? 2d * precision * recall / div : 0d;
}