I am trying to plot a bivariate ccdf
of the dataset that has x
and y
values both.
Univariate I can plot very well, below is the input and the code is for univeriate dataset.
Input: These are only first 20 rows of the data points. Input has 1000s of rows and of which col[1]
and col[3]
needs to be plotted as they posses a user and keyword frequency relationship.
tweetcricscore 34 #afgvssco 51
tweetcricscore 23 #afgvszim 46
tweetcricscore 24 #banvsire 12
tweetcricscore 456 #banvsned 46
tweetcricscore 653 #canvsnk 1
tweetcricscore 789 #cricket 178
tweetcricscore 625 #engvswi 46
tweetcricscore 86 #hkvssco 23
tweetcricscore 3 #indvsban 1
tweetcricscore 87 #sausvsvic 8
tweetcricscore 98 #wt20 56
Code: univeriate dataset
import numpy as np
import matplotlib.pyplot as plt
from pylab import*
import math
from matplotlib.ticker import LogLocator
data = np.genfromtxt('keyword.csv', delimiter=',', comments=None)
d0=data[:,1]
X0 = np.sort(d0)
cdf0 = np.arange(len(X0))/float(len(X0))
ccdf0 = 1 - cdf0
plt.plot(X0,ccdf0, color='b', marker='.', label='Keywords')
plt.legend(loc='upper right')
plt.xlabel('Freq (x)')
plt.ylabel('ccdf(x)')
plt.gca().set_xscale("log")
#plt.gca().set_yscale("log")
plt.show()
I am looking for some option for bivariate data points. I referred Seaborn Bivariate Distribution But I am not able to put it in proper context with my dataset.
Any alternative suggestion within python, matplotlib, seaborn are welcome..
Thanks in advance.
Bivariate distributions the way you're trying to describe are oftentimes continuous, for instance the size of a house (input, x) and it's price (output, y.) In your case there is no meaningful relationship (I think) in the number of the keyword, as it's probably just an ID assigned to the keyword right?
In your case to me it seems as though you have categories (keywords). each category appears to have two numbers a tweetcricscore
and a keyword
number. \
Your code here:
cdf0 = np.arange(len(X0))/float(len(X0))
To me suggests that your x range is just their labels and not a meaningful value.
A better source for categorical plots can be found here.
To create a bivariate distribution, assuming that's still what you want having read that, you'd do the following using your data as an example using your data from above:
import numpy as np
import seaborn as sns
col_1 = np.array([34, 23, 24, 456, 653, 789, 625, 86, 3, 87, 98])
col_3 = np.array([51, 46, 12, 46, 1, 178, 46, 23, 1, 8, 56])
sns.jointplot(x=col_3, y=col_1)
Which produces the very nonsensical figure here:
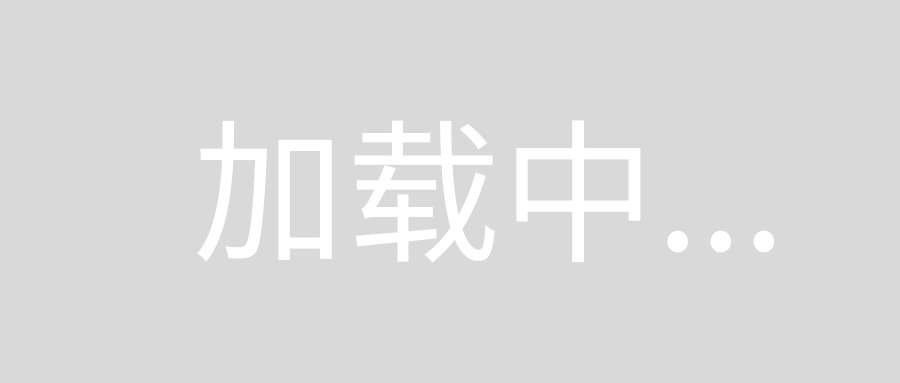
You'll have to add the x and y labels manually; this is because you're passing numpy
array
s instead of pandas
Dataframes
which can be thought of like dictionaries
where each key in the dictionary is the title of a column, and the value the numpy array.
Using random numbers to show how it might look with a more random, continuous, related dataset.
This is the example taken from the docs.
import numpy as np
import seaborn as sns
import pandas as pd
mean, cov = [0, 1], [(1, .5), (.5, 1)]
data = np.random.multivariate_normal(mean, cov, 200)
df = pd.DataFrame(data, columns=["x", "y"])
sns.jointplot(x="x", y="y", data=df);
Which gives this:
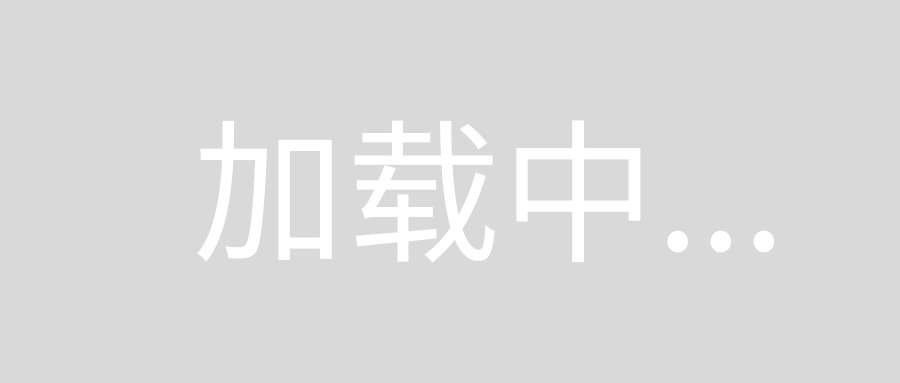
The bar graphs on top of the chart can be thought of as uni variate charts (what you probably have produced) because they just describe the distribution of one or the other variable (x, or y, col_3, or col_1)