I am interested in the Cholesky decomposition of large sparse matrices. The problem I'm having is that the Cholesky factors are not necessarily sparse (just like the product of two sparse matrices is not necessarily sparse).
For example for a matrix with non-zeros only along the first row, first column, and diagonal the Cholesky factors have 100% fill-in (the lower and upper triangles are 100% dense). In the image below the gray is non zero and the white is zero.
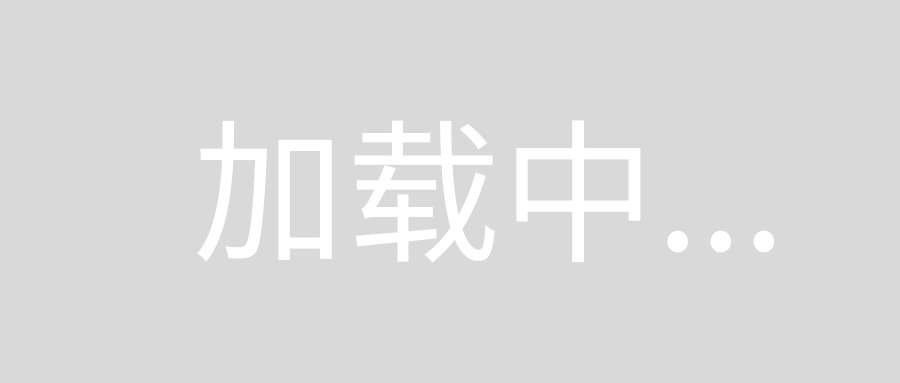
One solution I'm aware is to find a permutation P matrix and do the Cholesky decomposition of PTAP. For example with the same matrix by applying a permutation matrix which moves the first row to the last row and the first column to the last column the Cholesky factors are sparse.
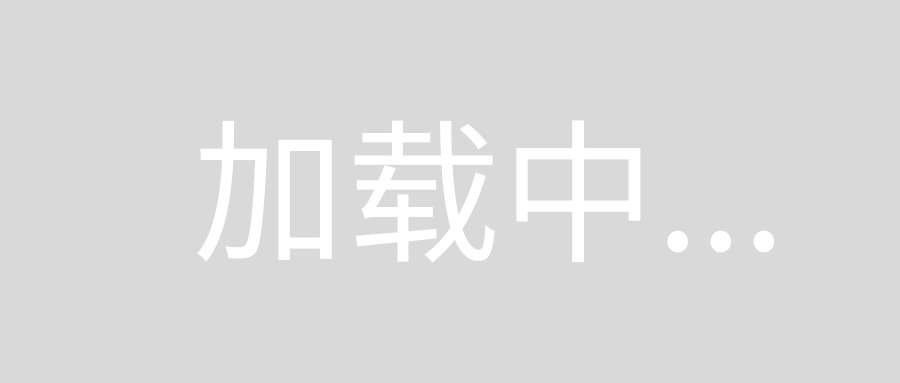
My question is how to determine P in general?
To get an idea of the difference of the Cholesky decomposition of A and PTAP from a more realistic matrix see the image below. I took all these images from http://www.seas.ucla.edu/~vandenbe/103/lectures/chol.pdf
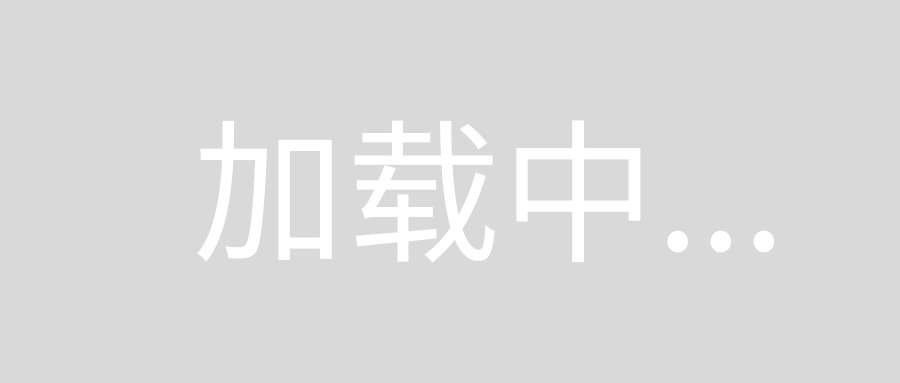
According to the lecture notes
many heuristic methods (that we don’t cover) exist for selecting good
permutation matrices P.
I would like to know what some of these methods are (code in C, C++, or even Java would be ideal).
The problem of finding an optimal permutation of rows and columns of a matrix for a minimum fill-in matrix-factorization is not a trivial trask (as pointed out in the comments). Thus, heuristic algorithms are used in praxis.
There are some libraries that implement heuristic renumbering/ordering-strategies, often based on graph-algorithms for the adjacency-graph of your matrix. One tries to reduce the bandwidth of the corresponding adjacency-matrix. An easy to implement algroithms is the Cuthill-McKee Algorithm or the Minimum-Degree Ordering algorithm. More about this problem can be found in the Book Yousef Saad: Iterative Methods for Sparse Linear Systems (2003), upon many others.
Many libraries implement heuristic algorithms, like
- Suitesparse A collection of libraries for direct solvers for largse sparse linear systems. Ordering methods implemented in the libraries AMD, CAMD, COLAMD, and CCOLAMD
- (Par-)Metis A library for Graph-partitioning, but provides Matrix reordering algorithms as well
-
Boost.Graph Working on the adjacency graph directly and provides some ordering algorithms, like the mentioned Cuthill-McKee, and Minimum-Degree Ordering
- (PT-)Scotch for Graph-partitioning and sparse-matrix reordering
Some of these libraries provide also sparse Cholesky factorization methods and can be used directly.