I would like to apply some basic clustering techniques to some latitude and longitude coordinates. Something along the lines of clustering (or some unsupervised learning) the coordinates into groups determined either by their great circle distance or their geodesic distance. NOTE: this could be a very poor approach, so please advise.
Ideally, I would like to tackle this in R
.
I have done some searching, but perhaps I missed a solid approach? I have come across the packages: flexclust
and pam
-- however, I have not come across a clear-cut example(s) with respect to the following:
- Defining my own distance function.
- Do either
flexclut
(via kcca
or cclust
) or pam
take into account random restarts?
- Icing on the cake = does anyone know of approaches/packages that would allow one to specify the minimum number of elements in each cluster?
Regarding your first question: Since the data is long/lat, one approach is to use earth.dist(...)
in package fossil
(calculates great circle dist):
library(fossil)
d = earth.dist(df) # distance object
Another approach uses distHaversine(...)
in the geosphere
package:
geo.dist = function(df) {
require(geosphere)
d <- function(i,z){ # z[1:2] contain long, lat
dist <- rep(0,nrow(z))
dist[i:nrow(z)] <- distHaversine(z[i:nrow(z),1:2],z[i,1:2])
return(dist)
}
dm <- do.call(cbind,lapply(1:nrow(df),d,df))
return(as.dist(dm))
}
The advantage here is that you can use any of the other distance algorithms in geosphere
, or you can define your own distance function and use it in place of distHaversine(...)
. Then apply any of the base R clustering techniques (e.g., kmeans, hclust):
km <- kmeans(geo.dist(df),centers=3) # k-means, 3 clusters
hc <- hclust(geo.dist(df)) # hierarchical clustering, dendrogram
clust <- cutree(hc, k=3) # cut the dendrogram to generate 3 clusters
Finally, a real example:
setwd("<directory with all files...>")
cities <- read.csv("GeoLiteCity-Location.csv",header=T,skip=1)
set.seed(123)
CA <- cities[cities$country=="US" & cities$region=="CA",]
CA <- CA[sample(1:nrow(CA),100),] # 100 random cities in California
df <- data.frame(long=CA$long, lat=CA$lat, city=CA$city)
d <- geo.dist(df) # distance matrix
hc <- hclust(d) # hierarchical clustering
plot(hc) # dendrogram suggests 4 clusters
df$clust <- cutree(hc,k=4)
library(ggplot2)
library(rgdal)
map.US <- readOGR(dsn=".", layer="tl_2013_us_state")
map.CA <- map.US[map.US$NAME=="California",]
map.df <- fortify(map.CA)
ggplot(map.df)+
geom_path(aes(x=long, y=lat, group=group))+
geom_point(data=df, aes(x=long, y=lat, color=factor(clust)), size=4)+
scale_color_discrete("Cluster")+
coord_fixed()
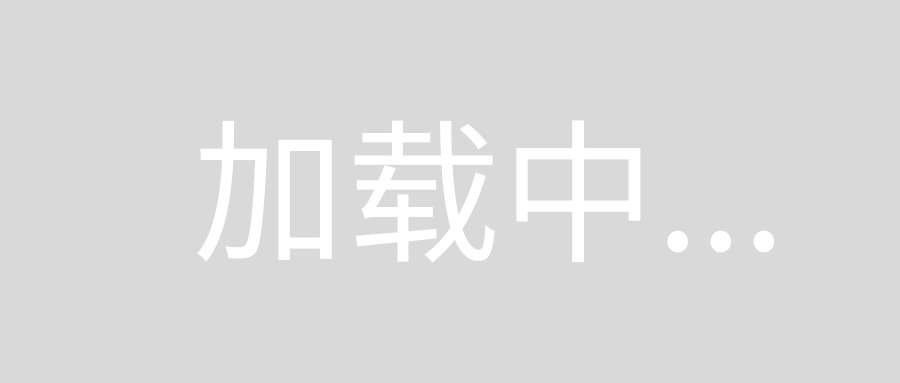
The city data is from GeoLite. The US States shapefile is from the Census Bureau.
Edit in response to @Anony-Mousse comment:
It may seem odd that "LA" is divided between two clusters, however, expanding the map shows that, for this random selection of cities, there is a gap between cluster 3 and cluster 4. Cluster 4 is basically Santa Monica and Burbank; cluster 3 is Pasadena, South LA, Long Beach, and everything south of that.
K-means clustering (4 clusters) does keep the area around LA/Santa Monica/Burbank/Long Beach in one cluster (see below). This just comes down to the different algorithms used by kmeans(...)
and hclust(...)
.
km <- kmeans(d, centers=4)
df$clust <- km$cluster
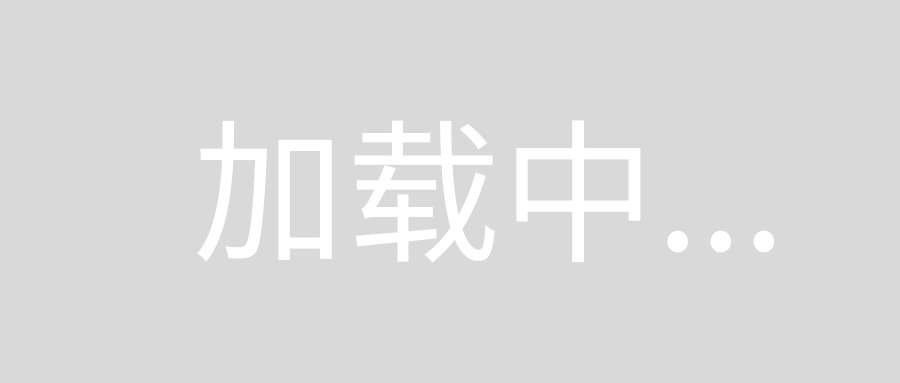
It's worth noting that these methods require that all points must go into some cluster. If you just ask which points are close together, and allow that some cities don't go into any cluster, you get very different results.
I've occasionally clustered spatial data with ELKI.
It's not R (I don't like R, and found it to be really slow in many situations. In fact, anything beyond simple matrix multiplications and simple calls into C- or Fortran code is slow.)
Anyway, ELKI has support for geodetic distances, and even index acceleration for these distances (both via the M-tree and the R*-tree; bulk-loaded R*-trees work best for me, and yield massive speedups); and many clustering algorithms such as DBSCAN and OPTICS can be used with these distance functions.
Here's an example of what I got with ELKI clustering: https://stackoverflow.com/a/14702758/1060350
I didn't keep the code though. Not sure whether I used Python for the KML output, or whether I implemented an ELKI output module.