I have grayscale images like this:
I want to detect anomalies on this kind of images. On the first image (upper-left) I want to detect three dots, on the second (upper-right) there is a small dot and a "Foggy area" (on the bottom-right), and on the last one, there is also a bit smaller dot somewhere in the middle of the image.
The normal static tresholding does't work ok for me, also Otsu's method is always the best choice. Is there any better, more robust or smarter way to detect anomalies like this? In Matlab I was using something like Frangi Filtering (eigenvalue filtering). Can anybody suggest good processing algorithm to solve anomaly detection on surfaces like this?
EDIT: Added another image with marked anomalies:
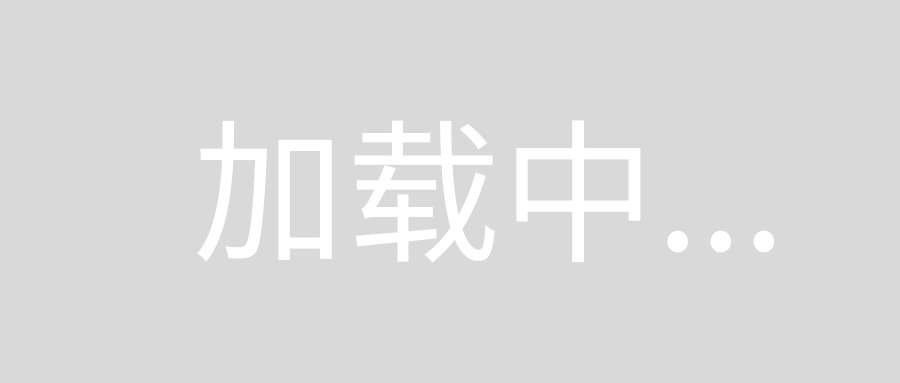
Using @Tapio 's tophat filtering and contrast adjustement.
Since @Tapio provide us with great idea how to increase contrast of anomalies on the surfaces like I asked at the begining, I provide all you guys with some of my results. I have and image like this:
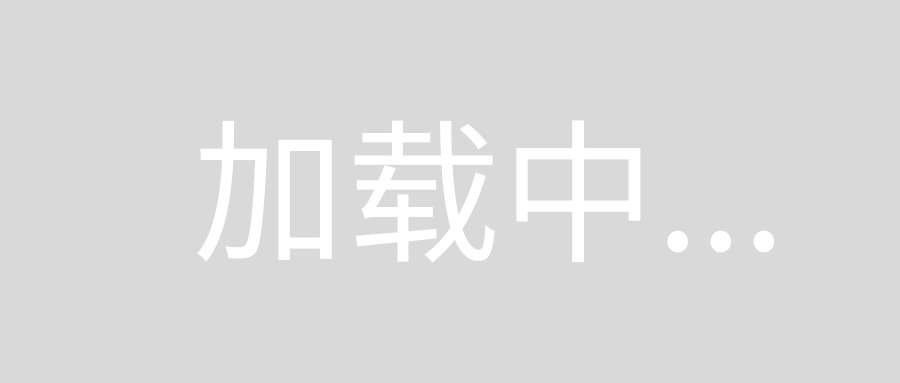
Here is my code how I use tophat filtering and contrast adjustement:
kernel = getStructuringElement(MORPH_ELLIPSE, Size(3, 3), Point(0, 0));
morphologyEx(inputImage, imgFiltered, MORPH_TOPHAT, kernel, Point(0, 0), 3);
imgAdjusted = imgFiltered * 7.2;
The result is here:
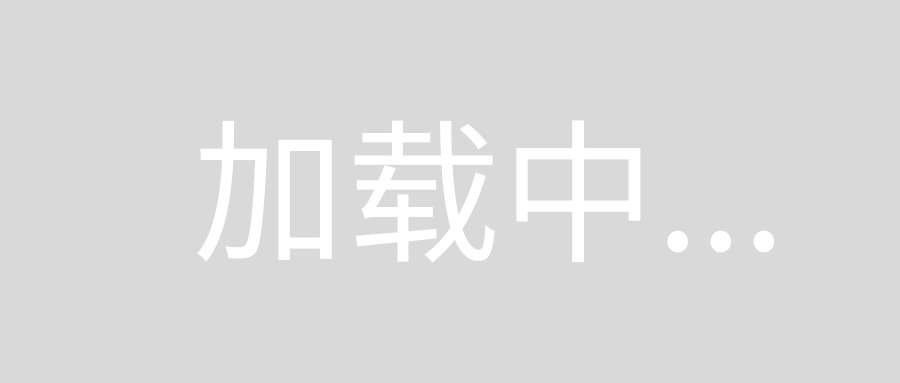
There is still question how to segment anomalies from the last image?? So if anybody have idea how to solve it, just take it! :) ??
You should take a look at bottom-hat filtering. It's defined as the difference of the original image and the morphological closing of the image and it makes small details such as the ones you are looking for flare out.
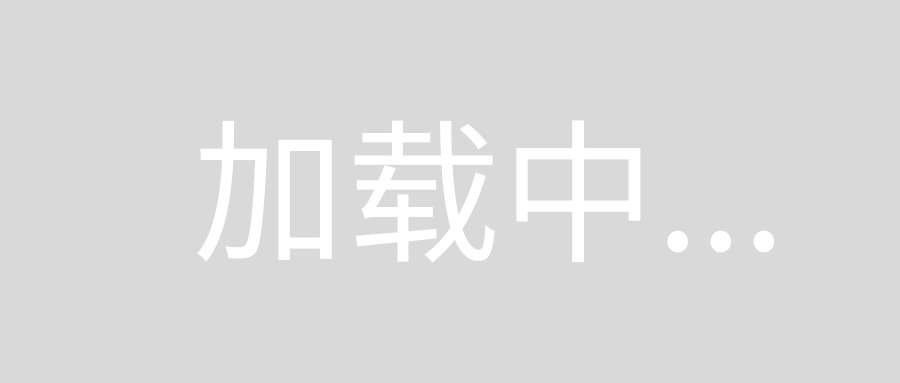
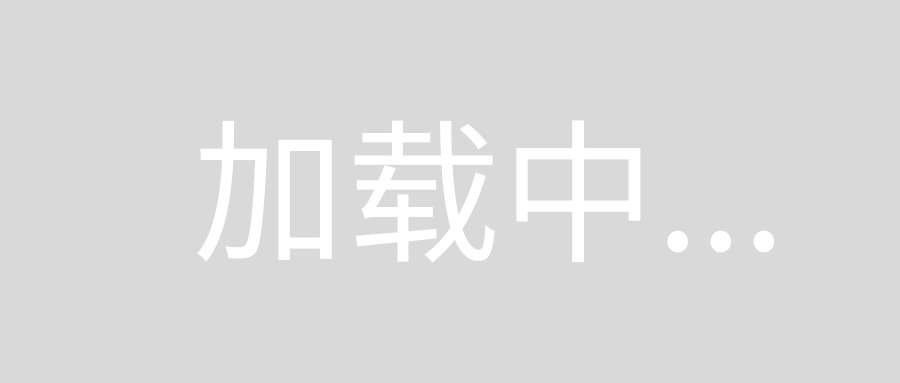
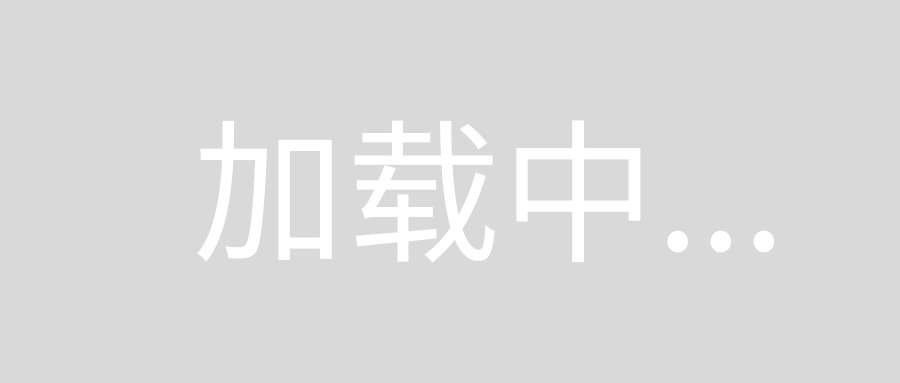
I adjusted the contrast to make both images visible. The anomalies are much more pronounced when looking at the intensities and are much easier to segment out.
Let's take a look at the first image:
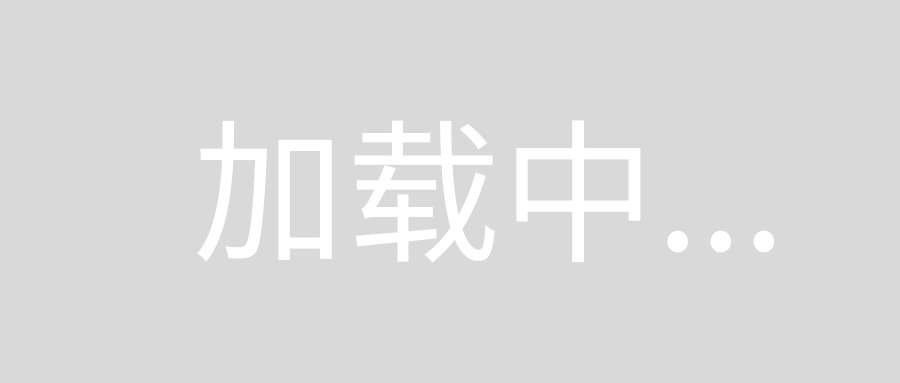
The histogram values don't represent the reality due to scaling caused by the visualization tools I'm using. However the relative distances do. So now the thresholding range is much larger, the target changed from a window to a barn door.
Global thresholding ( intensity > 15 ) :
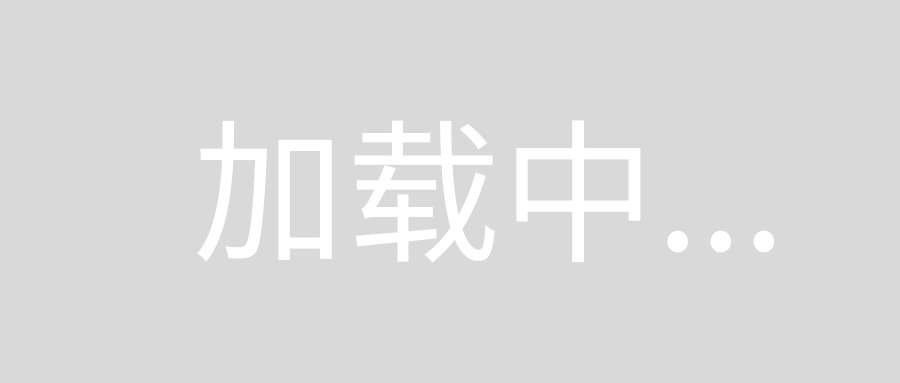
Otsu's method worked poorly here. It segmented all the small details to the foreground.
After removing noise by morphological opening :
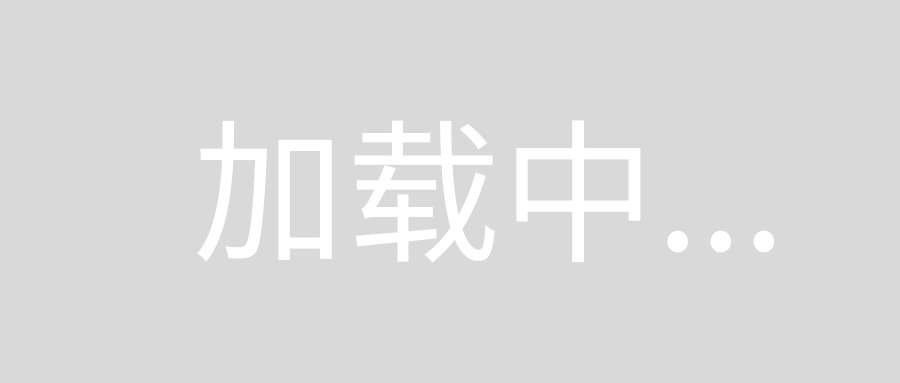
I also assumed that the black spots are the anomalies you are interested in. By setting the threshold lower you include more of the surface details. For example the third image does not have any particularly interesting features to my eye, but that's for you to judge. Like m3h0w said, it's a good heuristic to know that if something is hard for your eye to judge it's probably impossible for the computer.
@skoda23, I would try unsharp masking with fine tuned parameters for the blurring part, so that the high frequencies get emphasized and test it thoroughly so that no important information is lost in the process. Remember that it is usually not good idea to expect computer to do super-human work. If a human has doubts about where the anomalies are, computer will have to. Thus it is important to first preprocess the image, so that the anomalies are obvious for the human eye. Alternative for unsharp masking (or addition) might be CLAHE. But again: remember to fine tune it very carefully - it might bring out the texture of the board too much and interfere with your task.
Alternative approach to basic thresholding or Otsu's, would be AdaptiveThreshold()
which might be a good idea since there is a difference in intensity values between different regions you want to find.
My second guess would be first using fixed value thresholding for the darkest dots and then trying Sobel, or Canny. There should exist an optimal neighberhood where texture of the board will not shine as much and anomalies will. You can also try bluring before edge detection (if you've detected the small defects with the thresholding).
Again: it is vital for the task to experiment a lot on every step of this approach, because fine tuning the parameters will be crucial for eventual success. I'd recommend making friends with the trackbar, to speed up the process. Good luck!
You're basically dealing with the unfortunate fact that reality is analog. A threshold is a method to turn an analog range into a discrete (binary) range. Any threshold will do that. So what exactly do you mean with a "good enough" threshold?
Let's park that thought for a second. I see lots of anomalies - sort of thin grey worms. Apparently, you ignore them. I'm applying a different threshold then you are. This may be reasonable, but you're applying domain knowledge that I don't have.
I suspect these grey worms will be throwing off your fixed value thresholding. That's not to say the idea of a fixed threshold is bad. You can use it to find some artifacts and exclude those. Somewhat darkish patches will be missed, but can be brought out by replacing each pixel with the median value of its neighborhood, using a neighborhood size that's bigger than the width of those worms. In the dark patch, this does little, but it wipes out small local variations.
I don't pretend these two types of abnormalities are the only two, but that is really an application domain question and not about techniques. E.g. you don't appear to have ligthing artifacts (reflections), at least not in these 3 samples.